Publications
2023
Training Data Attribution for Diffusion Models
Diffusion models have become increasingly popular for synthesizing high-quality samples based on training datasets. However, given the oftentimes enormous sizes of the training datasets, it is difficult to assess how training data impact the samples produced by a trained diffusion model. The difficulty of relating diffusion model inputs and outputs poses significant challenges to model explainability and training data attribution. Here we propose a novel solution that reveals how training data influence the output of diffusion models through the use of ensembles. In our approach individual models in an encoded ensemble are trained on carefully engineered splits of the overall training data to permit the identification of influential training examples. The resulting model ensembles enable efficient ablation of training data influence, allowing us to assess the impact of training data on model outputs. We demonstrate the viability of these ensembles as generative models and the validity of our approach to assessing influence.

Zheng Dai, David Gifford
arXiv.
DOI: 10.48550/arXiv.2306.02174
Systematic elucidation of genetic mechanisms underlying cholesterol uptake
Genetic variation contributes greatly to LDL cholesterol (LDL-C) levels and coronary artery disease risk. By combining analysis of rare coding variants from the UK Biobank and genome-scale CRISPR-Cas9 knockout and activation screening, we substantially improve the identification of genes whose disruption alters serum LDL-C levels. We identify 21 genes in which rare coding variants significantly alter LDL-C levels at least partially through altered LDL-C uptake. We use co-essentiality-based gene module analysis to show that dysfunction of the RAB10 vesicle transport pathway leads to hypercholesterolemia in humans and mice by impairing surface LDL receptor levels. Further, we demonstrate that loss of function of OTX2 leads to robust reduction in serum LDL-C levels in mice and humans by increasing cellular LDL-C uptake. Altogether, we present an integrated approach that improves our understanding of the genetic regulators of LDL-C levels and provides a roadmap for further efforts to dissect complex human disease genetics.
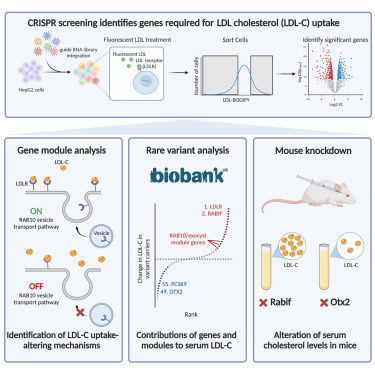
Marisa C. Hamilton, James D. Fife, Ersin Akinci, Tian Yu, Benyapa Khowpinitchai, Minsun Cha,Sammy Barkal,Thi Tun Thi,Grace H.T. Yeo,Juan Pablo Ramos Barroso,Matthew Jake Francoeur,Minja Velimirovic,David K. Gifford,Guillaume Lettre,Haojie Yu,Christopher A. Cassa,Richard I. Sherwood
Cell Genomics.
DOI: 10.1016/j.xgen.2023.100304
Fundamental Limits on the Robustness of Image Classifiers
We prove that image classifiers are fundamentally sensitive to small perturbations in their inputs. Specifically, we show that given some image space of n-by-n images, all but a tiny fraction of images in any image class induced over that space can be moved outside that class by adding some perturbation whose p-norm is O(n 1/ max (p,1)), as long as that image class takes up at most half of the image space. We then show that O(n 1/ max (p,1)) is asymptotically optimal. Finally, we show that an increase in the bit depth of the image space leads to a loss in robustness. We supplement our results with a discussion of their implications for vision systems.
Short introductory talk (4:35) can be found here (linked to Vimeo).
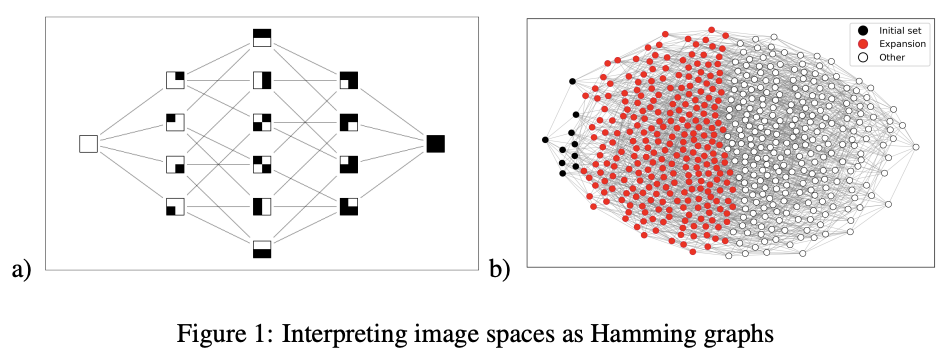
Zheng Dai, David Gifford
ICLR.
A pan-variant mRNA-LNP T cell vaccine protects HLA transgenic mice from mortality after infection with SARS-CoV-2 Beta
Licensed COVID-19 vaccines ameliorate viral infection by inducing production of neutralizing antibodies that bind the SARS-CoV-2 Spike protein and inhibit viral cellular entry. However, the clinical effectiveness of these vaccines is transitory as viral variants escape antibody neutralization. Effective vaccines that solely rely upon a T cell response to combat SARS-CoV-2 infection could be transformational because they can utilize highly conserved short pan-variant peptide epitopes, but a mRNA-LNP T cell vaccine has not been shown to provide effective anti-SARS-CoV-2 prophylaxis. Here we show a mRNA-LNP vaccine (MIT-T-COVID) based on highly conserved short peptide epitopes activates CD8+ and CD4+ T cell responses that attenuate morbidity and prevent mortality in HLA-A 02:01 transgenic mice infected with SARS-CoV-2 Beta (B.1.351). We found CD8+ T cells in mice immunized with MIT-T-COVID vaccine significantly increased from 1.1% to 24.0% of total pulmonary nucleated cells prior to and at 7 days post infection (dpi), respectively, indicating dynamic recruitment of circulating specific T cells into the infected lungs. Mice immunized with MIT-T-COVID had 2.8 (2 dpi) and 3.3 (7 dpi) times more lung infiltrating CD8+ T cells than unimmunized mice. Mice immunized with MIT-T-COVID had 17.4 times more lung infiltrating CD4+ T cells than unimmunized mice (7 dpi). The undetectable specific antibody response in MIT-T-COVID-immunized mice demonstrates specific T cell responses alone can effectively attenuate the pathogenesis of SARS-CoV-2 infection. Our results suggest further study is merited for pan-variant T cell vaccines, including for individuals that cannot produce neutralizing antibodies or to help mitigate Long COVID.
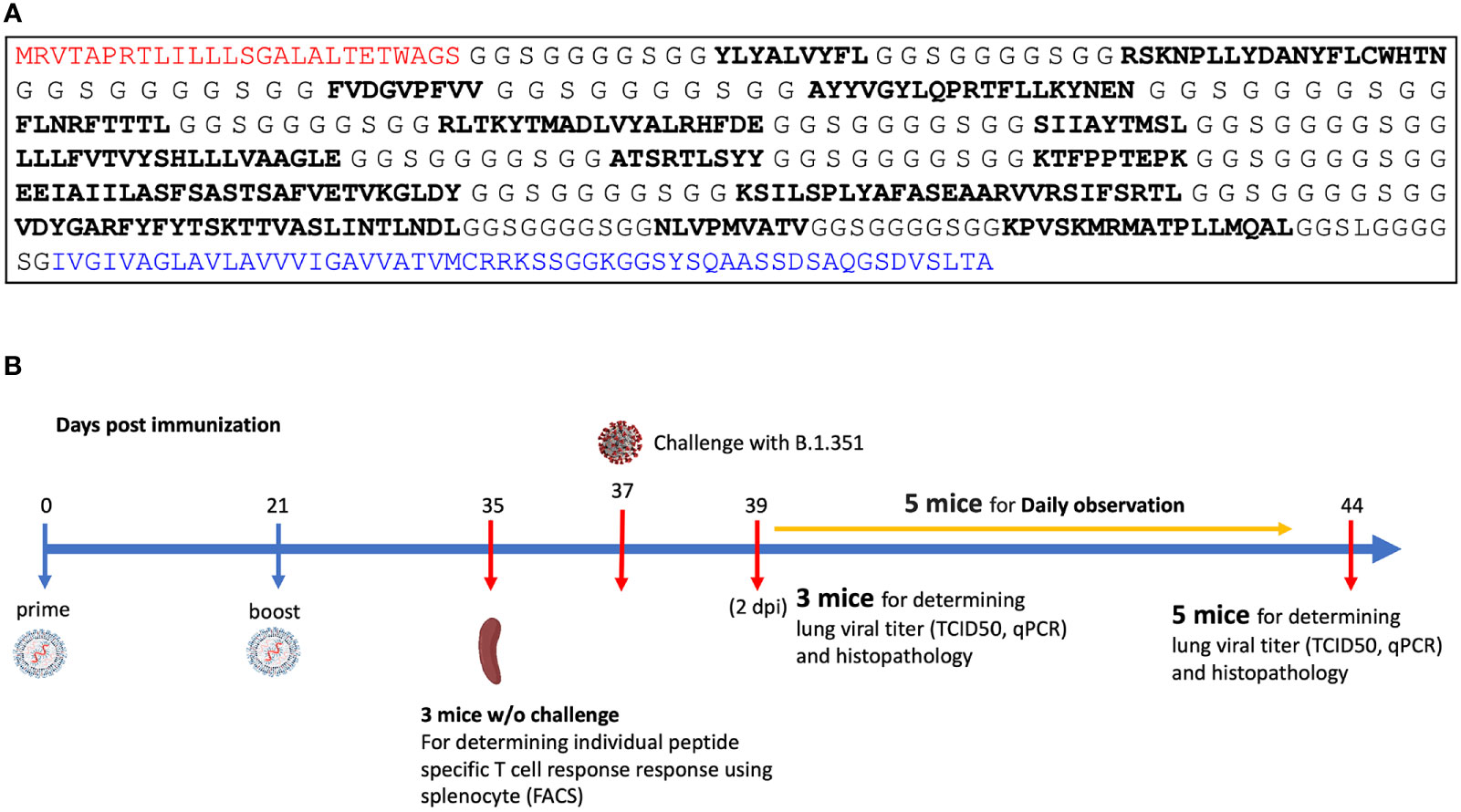
Brandon Carter, Pinghan Huang, Ge Liu, David Gifford
Frontiers in Immunology.
DOI: 10.3389/fimmu.2023.1135815
Constrained Submodular Optimization for Vaccine Design
Advances in machine learning have enabled the prediction of immune system responses to prophylactic and therapeutic vaccines. However, the engineering task of designing vaccines remains a challenge. In particular, the genetic variability of the human immune system makes it difficult to design peptide vaccines that provide widespread immunity in vaccinated populations. We introduce a framework for evaluating and designing peptide vaccines that uses probabilistic machine learning models, and demonstrate its ability to produce designs for a SARS-CoV-2 vaccine that outperform previous designs. We provide a theoretical analysis of the approximability, scalability, and complexity of our framework.
Short introductory talk (14:07) can be found here (linked to Vimeo).
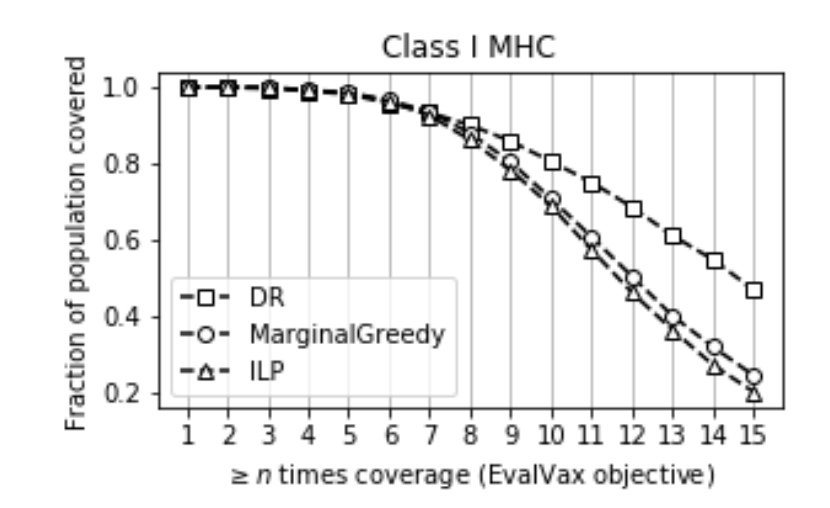
Zheng Dai, David Gifford
AAAI.
DOI: 10.48550/arXiv.2206.08336
2022
Computational counterselection identifies nonspecific therapeutic biologic candidates
Effective biologics require high specificity and limited off-target binding, but these properties are not guaranteed by current affinity-selection-based discovery methods. Molecular counterselection against off targets is a technique for identifying nonspecific sequences but is experimentally costly and can fail to eliminate a large fraction of nonspecific sequences. Here, we introduce computational counterselection, a framework for removing nonspecific sequences from pools of candidate biologics using machine learning models. We demonstrate the method using sequencing data from single-target affinity selection of antibodies, bypassing combinatorial experiments. We show that computational counterselection outperforms molecular counterselection by performing cross-target selection and individual binding assays to determine the performance of each method at retaining on-target, specific antibodies and identifying and eliminating off-target, nonspecific antibodies. Further, we show that one can identify generally polyspecific antibody sequences using a general model trained on affinity data from unrelated targets with potential affinity for a broad range of sequences.
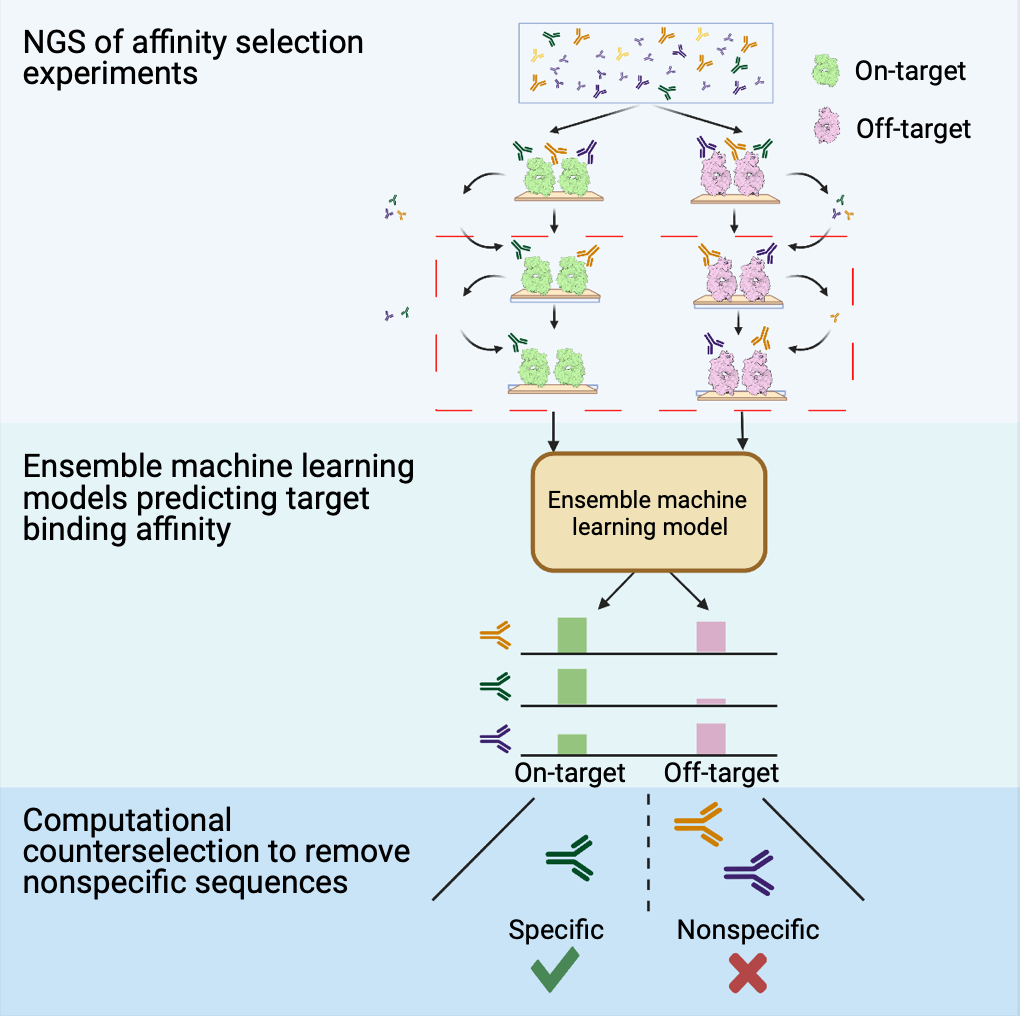
Sachit Dinesh Saksena, Ge Liu, Christine Banholzer, Geraldine Horny,Stefan Ewert, David K. Gifford
Cell Reports Methods.
DOI: 10.1016/j.crmeth.2022.100254
Ultra high diversity factorizable libraries for efficient therapeutic discovery
The successful discovery of novel biological therapeutics by selection requires highly diverse libraries of candidate sequences that contain a high proportion of desirable candidates. Here we propose the use of computationally designed factorizable libraries made of concatenated segment libraries as a method of creating large libraries that meet an objective function at low cost. We show that factorizable libraries can be designed efficiently by representing objective functions that describe sequence optimality as an inner product of feature vectors, which we use to design an optimization method we call Stochastically Annealed Product Spaces (SAPS). We then use this approach to design diverse and efficient libraries of antibody CDR-H3 sequences with various optimized characteristics.
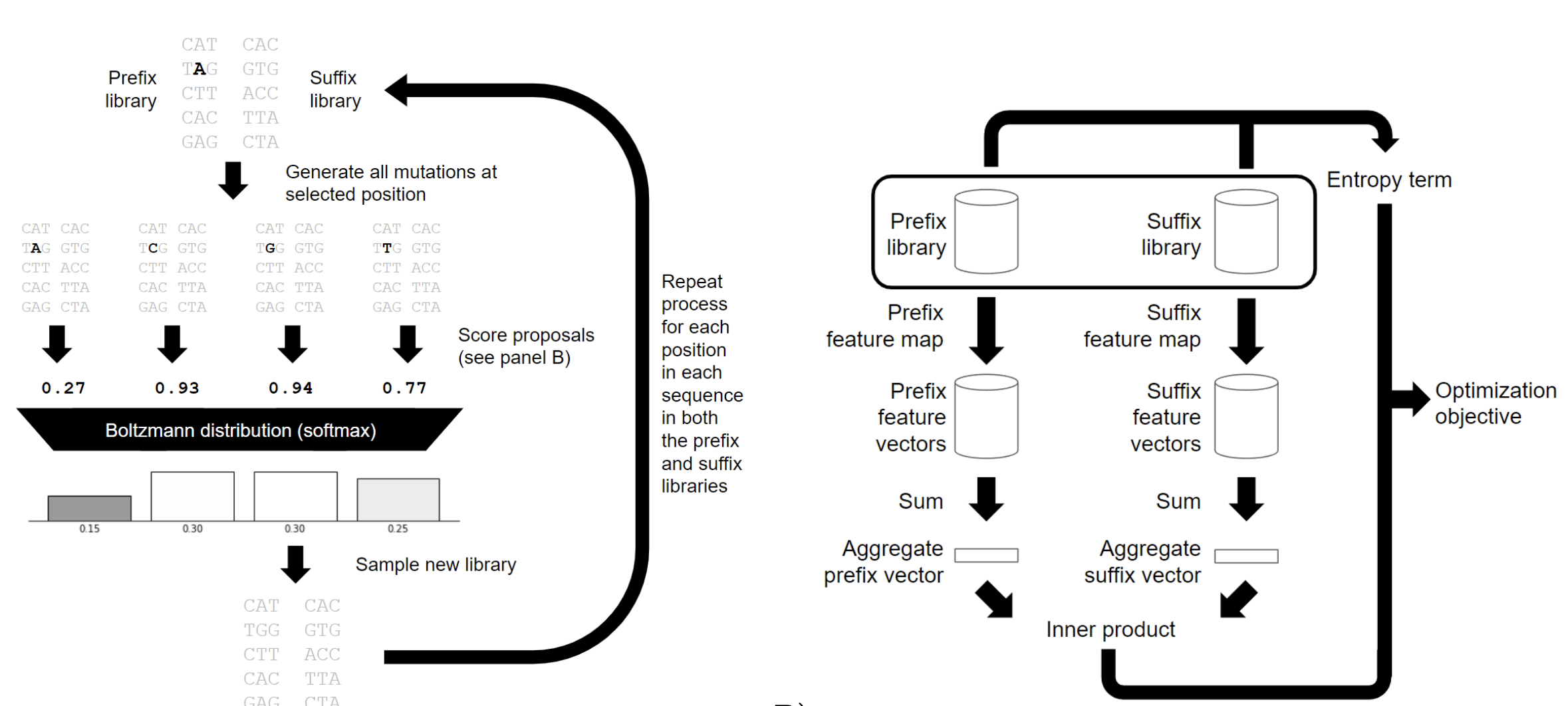
Zheng Dai*, Sachit D. Saksena*, Geraldine Horny, Christine Banholzer, Stefan Ewert, David K. Gifford
Genome Research. 1088-9051
DOI: 10.1101/gr.276593.122
Ranking reprogramming factors for cell differentiation
Transcription factor over-expression is a proven method for reprogramming cells to a desired cell type for regenerative medicine and therapeutic discovery. However, a general method for the identification of reprogramming factors to create an arbitrary cell type is an open problem. Here we examine the success rate of methods and data for differentiation by testing the ability of nine computational methods (CellNet, GarNet, EBseq, AME, DREME, HOMER, KMAC, diffTF and DeepAccess) to discover and rank candidate factors for eight target cell types with known reprogramming solutions. We compare methods that use gene expression, biological networks and chromatin accessibility data, and comprehensively test parameter and preprocessing of input data to optimize performance. We find the best factor identification methods can identify an average of 50–60% of reprogramming factors within the top ten candidates, and methods that use chromatin accessibility perform the best. Among the chromatin accessibility methods, complex methods DeepAccess and diffTF have higher correlation with the ranked significance of transcription factor candidates within reprogramming protocols for differentiation. We provide evidence that AME and diffTF are optimal methods for transcription factor recovery that will allow for systematic prioritization of transcription factor candidates to aid in the design of new reprogramming protocols.
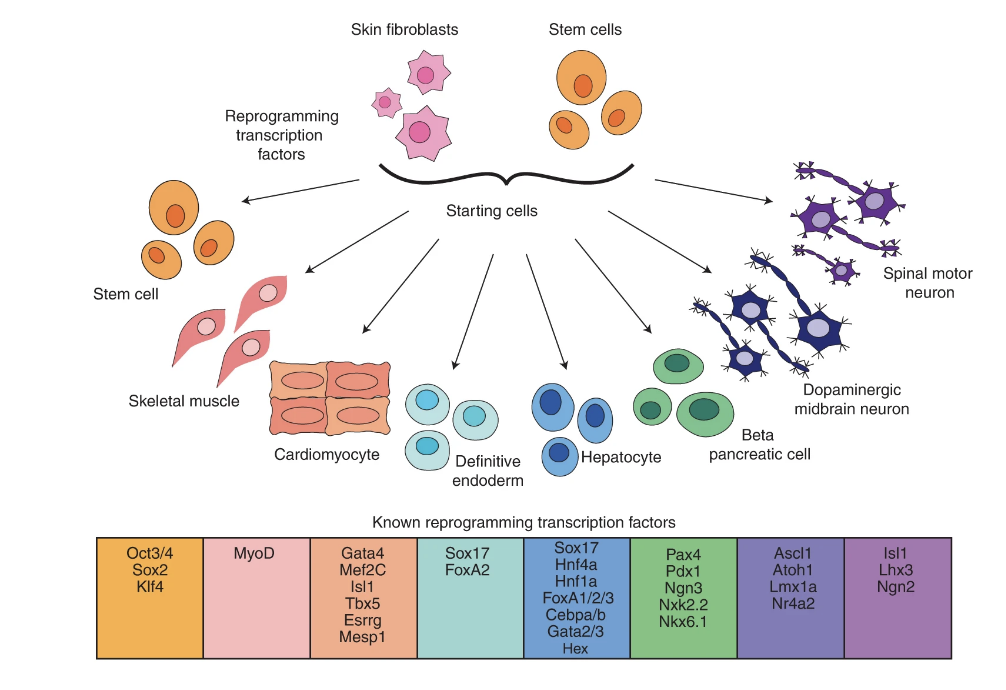
Jennifer Hammelman, Tulsi Patel, Michael Closser, Hynek Wichterle, David Gifford
Nature Methods.
DOI: 10.1038/s41592-022-01522-2
Maximum n-times Coverage for Vaccine Design
We introduce the maximum n-times coverage problem that selects k overlays to maximize the summed coverage of weighted elements, where each element must be covered at least n times. We also define the min-cost n-times coverage problem where the objective is to select the minimum set of overlays such that the sum of the weights of elements that are covered at least n times is at least τ. Maximum n-times coverage is a generalization of the multi-set multi-cover problem, is NP-complete, and is not submodular. We introduce two new practical solutions for n-times coverage based on integer linear programming and sequential greedy optimization. We show that maximum n-times coverage is a natural way to frame peptide vaccine design, and find that it produces a pan-strain COVID-19 vaccine design that is superior to 29 other published designs in predicted population coverage and the expected number of peptides displayed by each individual’s HLA molecules.
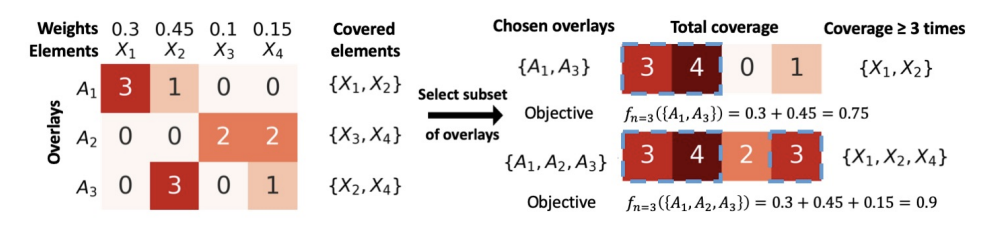
Ge Liu, Alexander Dimitrakakis, Brandon Carter, David Gifford
International Conference on Learning Representations. preprint arXiv:2101.10902
arXiv: 2101.10902
2021
Image classifiers can not be made robust to small perturbations
The sensitivity of image classifiers to small perturbations in the input is often viewed as a defect of their construction. We demonstrate that this sensitivity is a fundamental property of classifiers. For any arbitrary classifier over the set of n-by-n images, we show that for all but one class it is possible to change the classification of all but a tiny fraction of the images in that class with a tiny modification compared to the diameter of the image space when measured in any p-norm, including the hamming distance. We then examine how this phenomenon manifests in human visual perception and discuss its implications for the design considerations of computer vision systems.
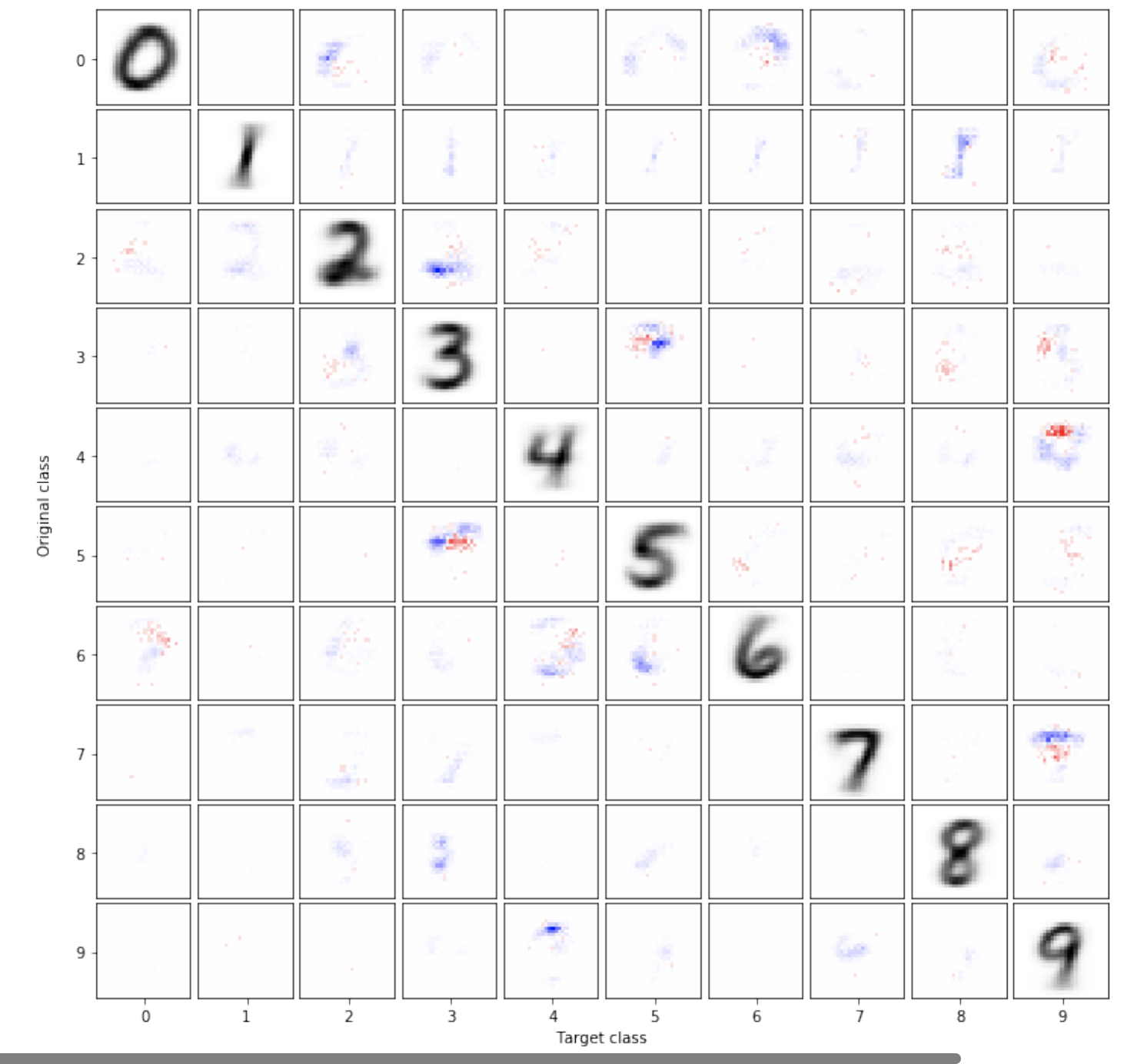
Zheng Dai, David K Gifford
arXiv. Volume 37, Issue 19
DOI: 10.48550/arXiv.2112.04033
Overinterpretation reveals image classification model pathologies
Image classifiers are typically scored on their test set accuracy, but high accuracy can mask a subtle type of model failure. We find that high scoring convolutional neural networks (CNNs) on popular benchmarks exhibit troubling pathologies that allow them to display high accuracy even in the absence of semantically salient features. When a model provides a high-confidence decision without salient supporting input features, we say the classifier has overinterpreted its input, finding too much class-evidence in patterns that appear nonsensical to humans. Here, we demonstrate that neural networks trained on CIFAR-10 and ImageNet suffer from overinterpretation, and we find models on CIFAR-10 make confident predictions even when 95% of input images are masked and humans cannot discern salient features in the remaining pixel-subsets. We introduce Batched Gradient SIS, a new method for discovering sufficient input subsets for complex datasets, and use this method to show the sufficiency of border pixels in ImageNet for training and testing. Although these patterns portend potential model fragility in real-world deployment, they are in fact valid statistical patterns of the benchmark that alone suffice to attain high test accuracy. Unlike adversarial examples, overinterpretation relies upon unmodified image pixels. We find ensembling and input dropout can each help mitigate overinterpretation.
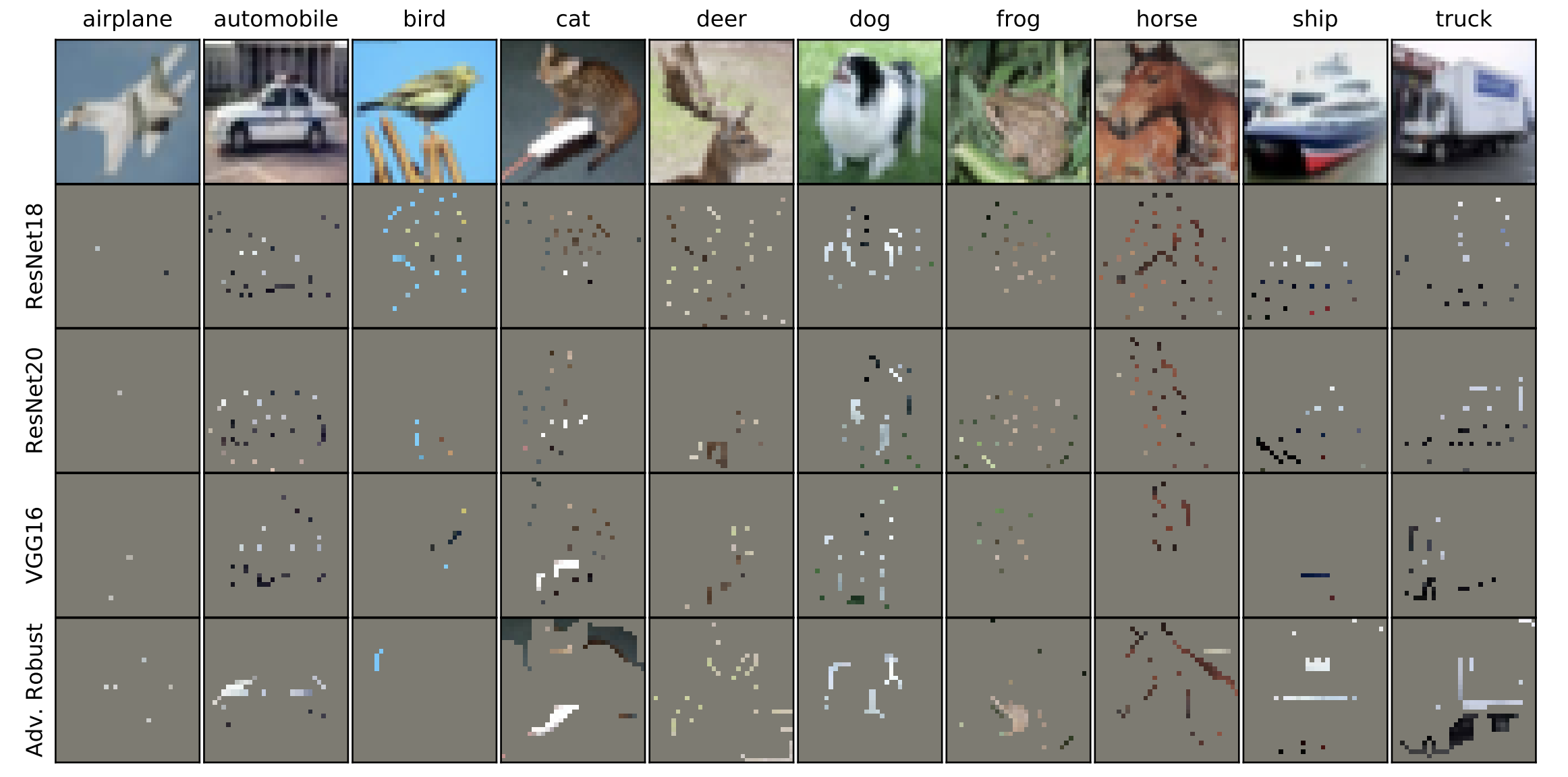
Brandon Carter, Siddhartha Jain, Jonas Mueller and David Gifford
Conference on Neural Information Processing Systems. preprint arXiv:2003.08907
arXiv: 2003.08907
Discovering differential genome sequence activity with interpretable and efficient deep learning
Discovering sequence features that differentially direct cells to alternate fates is key to understanding both cellular development and the consequences of disease related mutations. We introduce Expected Pattern Effect and Differential Expected Pattern Effect, two black-box methods that can interpret genome regulatory sequences for cell type-specific or condition specific patterns. We show that these methods identify relevant transcription factor motifs and spacings that are predictive of cell state-specific chromatin accessibility. Finally, we integrate these methods into framework that is readily accessible to non-experts and available for download as a binary or installed via PyPI or bioconda at https://cgs.csail.mit.edu/deepaccess-package/.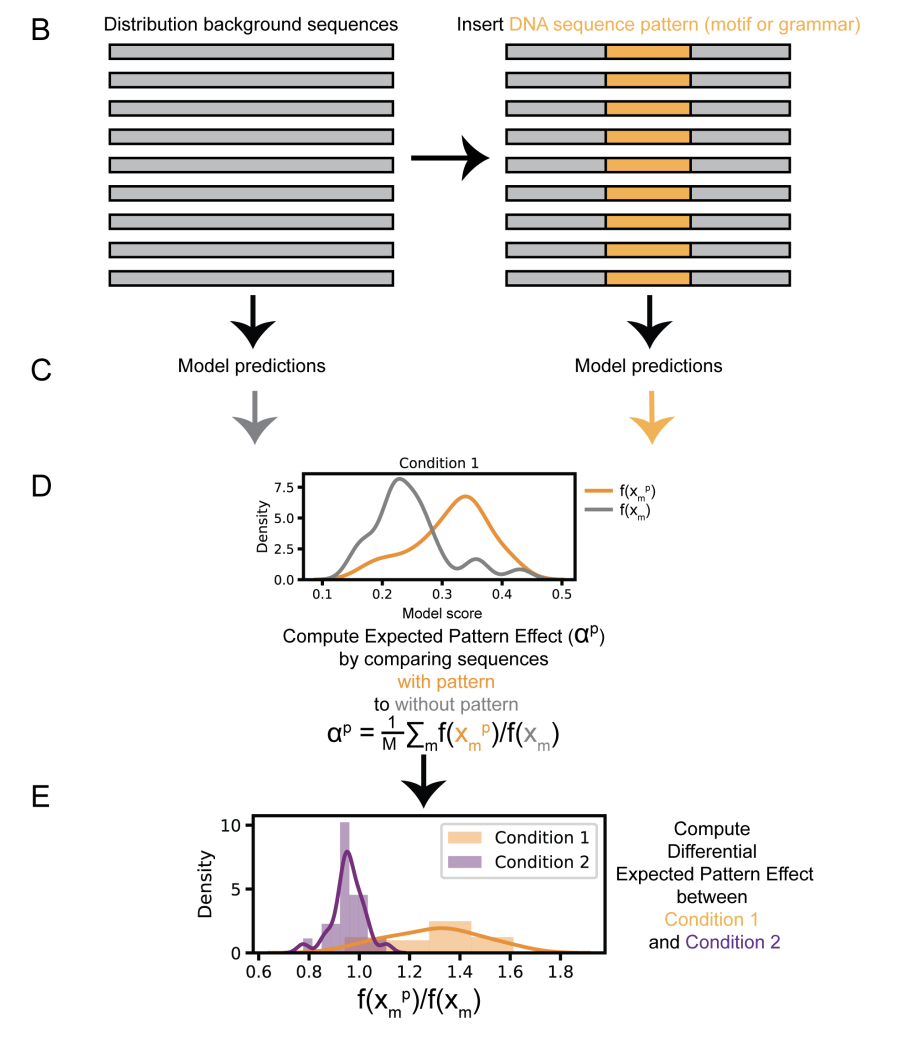
Jennifer Hammelman,David K. Gifford
PLOS Computational Biology.
DOI: 10.1371/journal.pcbi.1009282
seqgra: Principled Selection of Neural Network Architectures for Genomics Prediction Tasks
Sequence models based on deep neural networks have achieved state-of-the-art performance on regulatory genomics prediction tasks, such as chromatin accessibility and transcription factor binding. But despite their high accuracy, their contributions to a mechanistic understanding of the biology of regulatory elements is often hindered by the complexity of the predictive model and thus poor interpretability of its decision boundaries. To address this, we introduce seqgra, a deep learning pipeline that incorporates the rule-based simulation of biological sequence data and the training and evaluation of models, whose decision boundaries mirror the rules from the simulation process. The method can be used to (1) generate data under the assumption of a hypothesized model of genome regulation, (2) identify neural network architectures capable of recovering the rules of said model, and (3) analyze a model’s predictive performance as a function of training set size, noise level, and the complexity of the rules behind the simulated data.
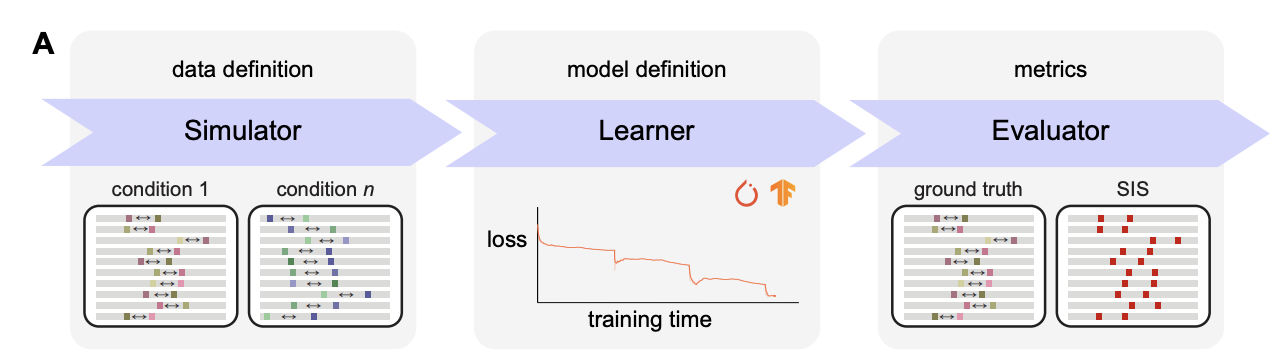
Konstantin Krismer, Jennifer Hammelman, David K. Gifford
bioRxiv.
DOI: 10.1101/2021.06.14.448415
Generative modeling of single-cell time series with PRESCIENT enables prediction of cell trajectories with interventions
Existing computational methods that use single-cell RNA-sequencing (scRNA-seq) for cell fate prediction do not model how cells evolve stochastically and in physical time, nor can they predict how differentiation trajectories are altered by proposed interventions. We introduce PRESCIENT (Potential eneRgy undErlying Single Cell gradIENTs), a generative modeling framework that learns an underlying differentiation landscape from time-series scRNA-seq data. We validate PRESCIENT on an experimental lineage tracing dataset, where we show that PRESCIENT is able to predict the fate biases of progenitor cells in hematopoiesis when accounting for cell proliferation, improving upon the best-performing existing method. We demonstrate how PRESCIENT can simulate trajectories for perturbed cells, recovering the expected effects of known modulators of cell fate in hematopoiesis and pancreatic β cell differentiation. PRESCIENT is able to accommodate complex perturbations of multiple genes, at different time points and from different starting cell populations, and is available at https://github.com/gifford-lab/prescient.
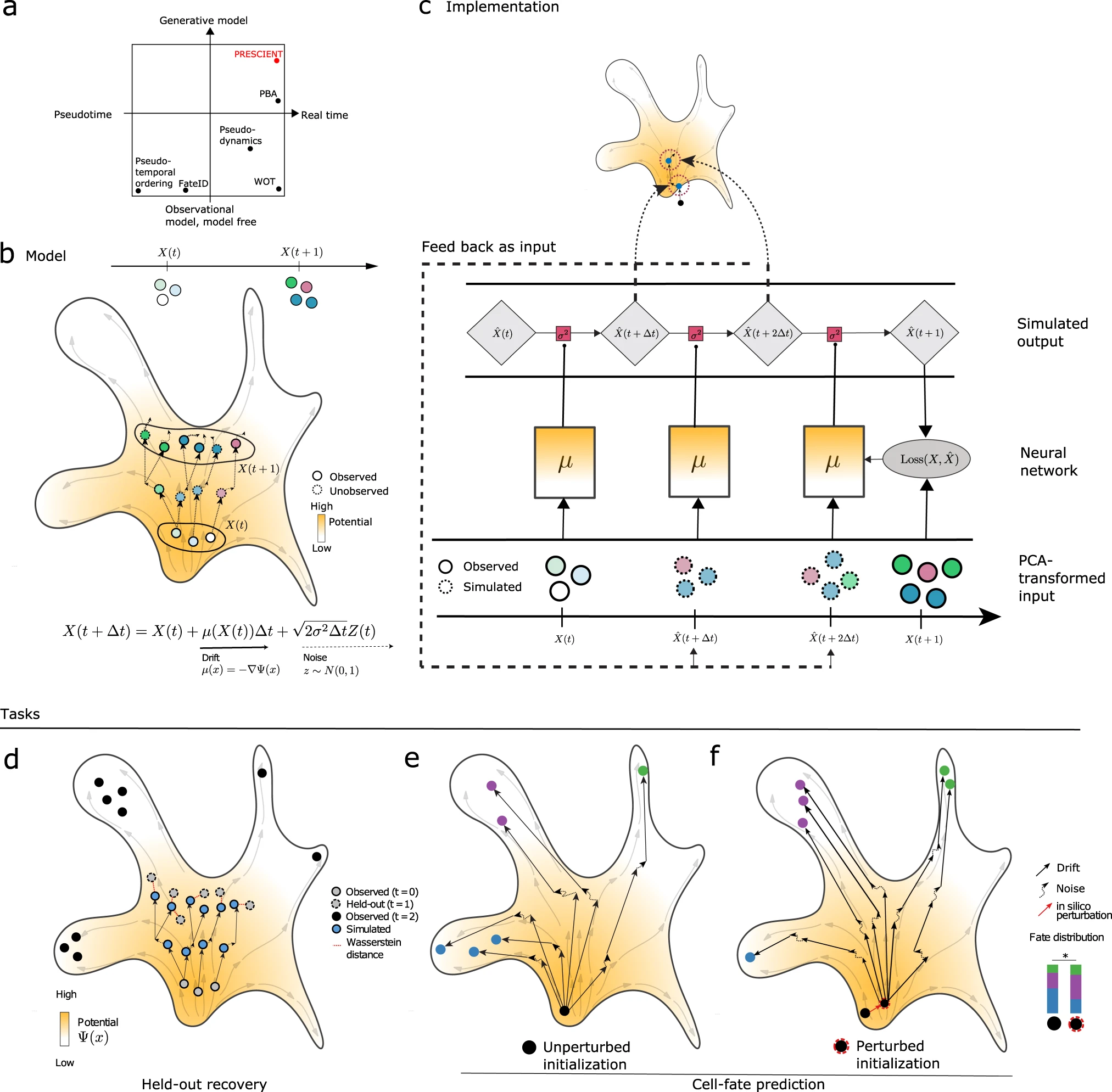
Grace Hui Ting Yeo*, Sachit D. Saksena*, and David K. Gifford
Nature Communications. 12, 3222 (2021)
DOI: 10.1038/s41467-021-23518-w
spatzie: An R package for identifying significant transcription factor motif co-enrichment from enhancer-promoter interactions
Genomic interactions provide important context to our understanding of the state of the genome. One question is whether specific transcription factor interactions give rise to genome organization. We introduce spatzie, an R package and a website that implements statistical tests for significant transcription factor motif cooperativity between enhancer-promoter interactions. We conducted controlled experiments under realistic simulated data from ChIP-seq to confirm spatzie is capable of discovering co-enriched motif interactions even in noisy conditions. We then use spatzie to investigate cell type specific transcription factor cooperativity within recent human ChIA-PET enhancer-promoter interaction data. The method is available online at https://spatzie.mit.edu.
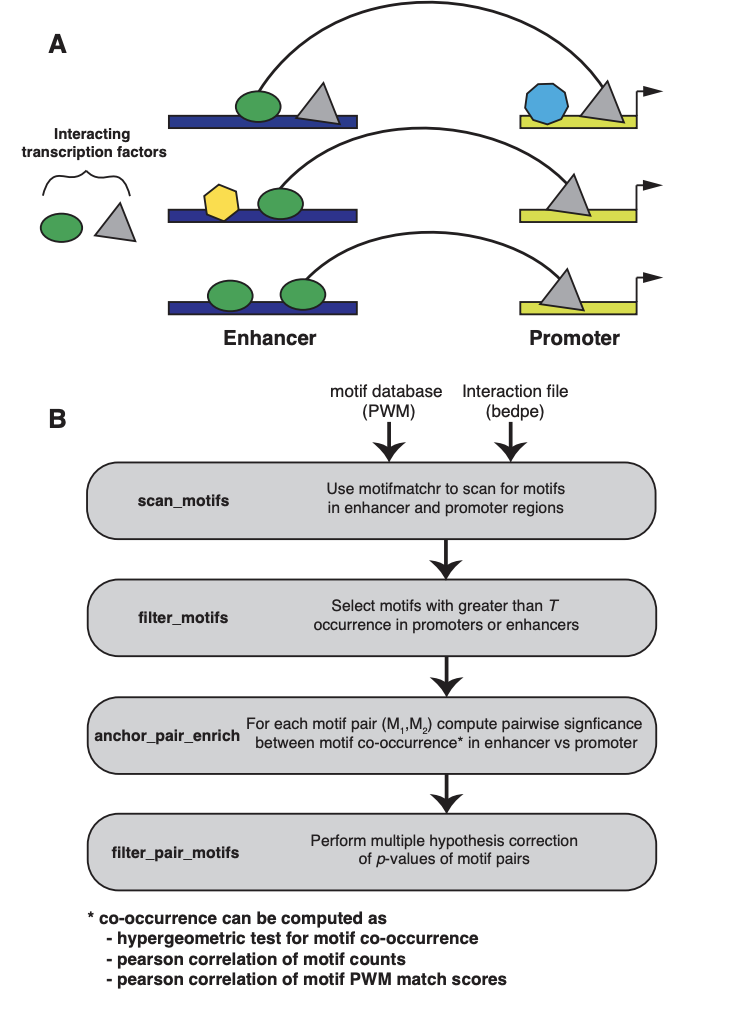
Jennifer Hammelman, Konstantin Krismer, David K. Gifford
bioRxiv.
DOI: 10.1101/2021.05.25.445606
Detection of gene cis-regulatory element perturbations in single-cell transcriptomes
We introduce poly-adenine CRISPR gRNA-based single-cell RNA-sequencing (pAC-Seq), a method that enables the direct observation of guide RNAs (gRNAs) in scRNA-seq. We use pAC-Seq to assess the phenotypic consequences of CRISPR/Cas9 based alterations of gene cis-regulatory regions. We show that pAC-Seq is able to detect cis-regulatory-induced alteration of target gene expression even when biallelic loss of target gene expression occurs in only ~5% of cells. This low rate of biallelic loss significantly increases the number of cells required to detect the consequences of changes to the regulatory genome, but can be ameliorated by transcript-targeted sequencing. Based on our experimental results we model the power to detect regulatory genome induced transcriptomic effects based on the rate of mono/biallelic loss, baseline gene expression, and the number of cells per target gRNA.
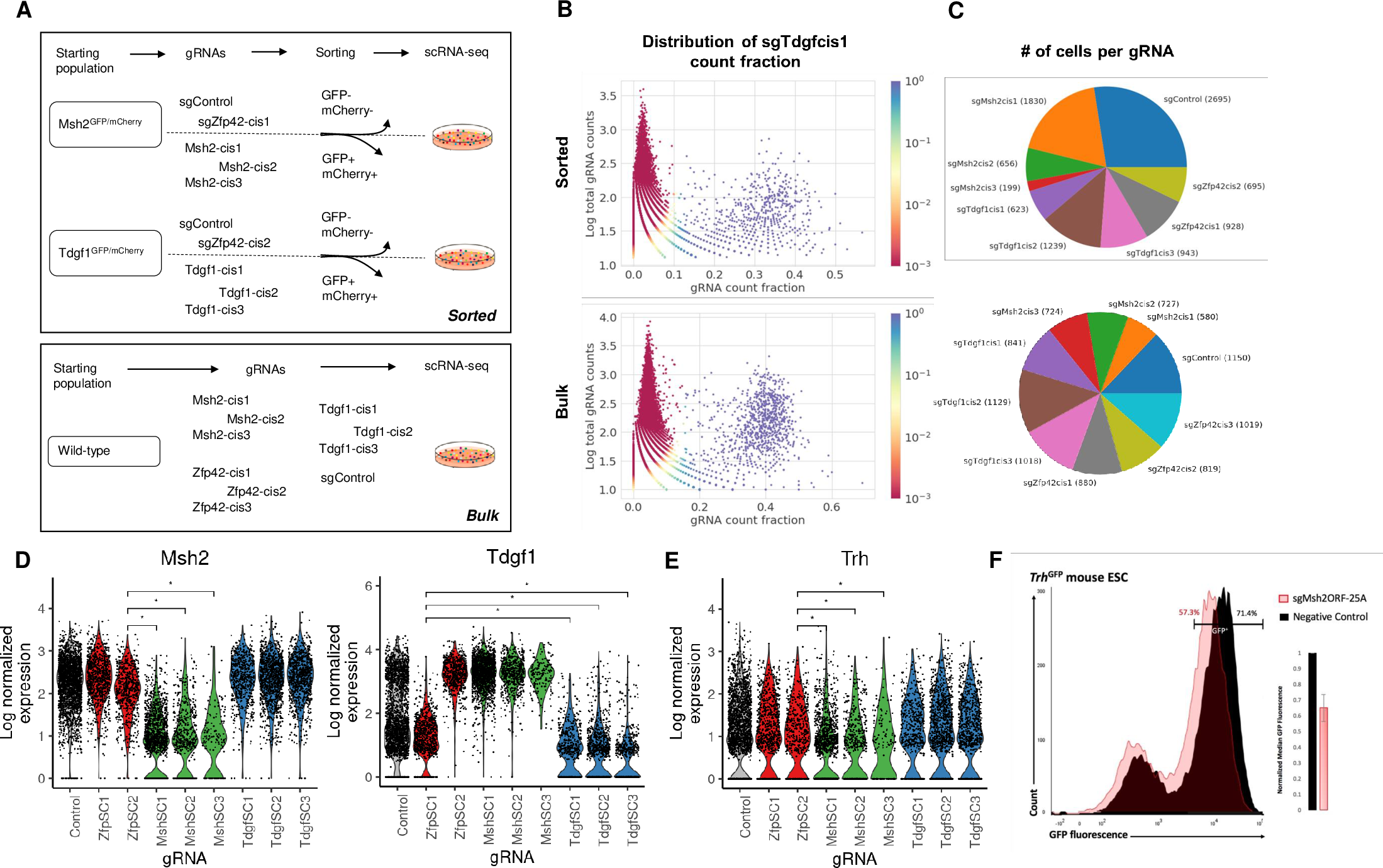
Grace Hui Ting Yeo, Oscar Juez, Qing Chen, Budhaditya Banerjee, Lendy Chu, Max W. Shen, May Sabry, Ive Logister, Richard I. Sherwood, David K. Gifford
PLoS Computational Biology. Volume 17, Issue 3, e1008789
DOI: 10.1371/journal.pcbi.1008789
Machine learning optimization of peptides for presentation by class II MHCs
T cells play a critical role in cellular immune responses to pathogens and cancer and can be activated and expanded by Major Histocompatibility Complex (MHC)-presented antigens contained in peptide vaccines. We present a machine learning method to optimize the presentation of peptides by class II MHCs by modifying their anchor residues. Our method first learns a model of peptide affinity for a class II MHC using an ensemble of deep residual networks, and then uses the model to propose anchor residue changes to improve peptide affinity. We use a high throughput yeast display assay to show that anchor residue optimization improves peptide binding.
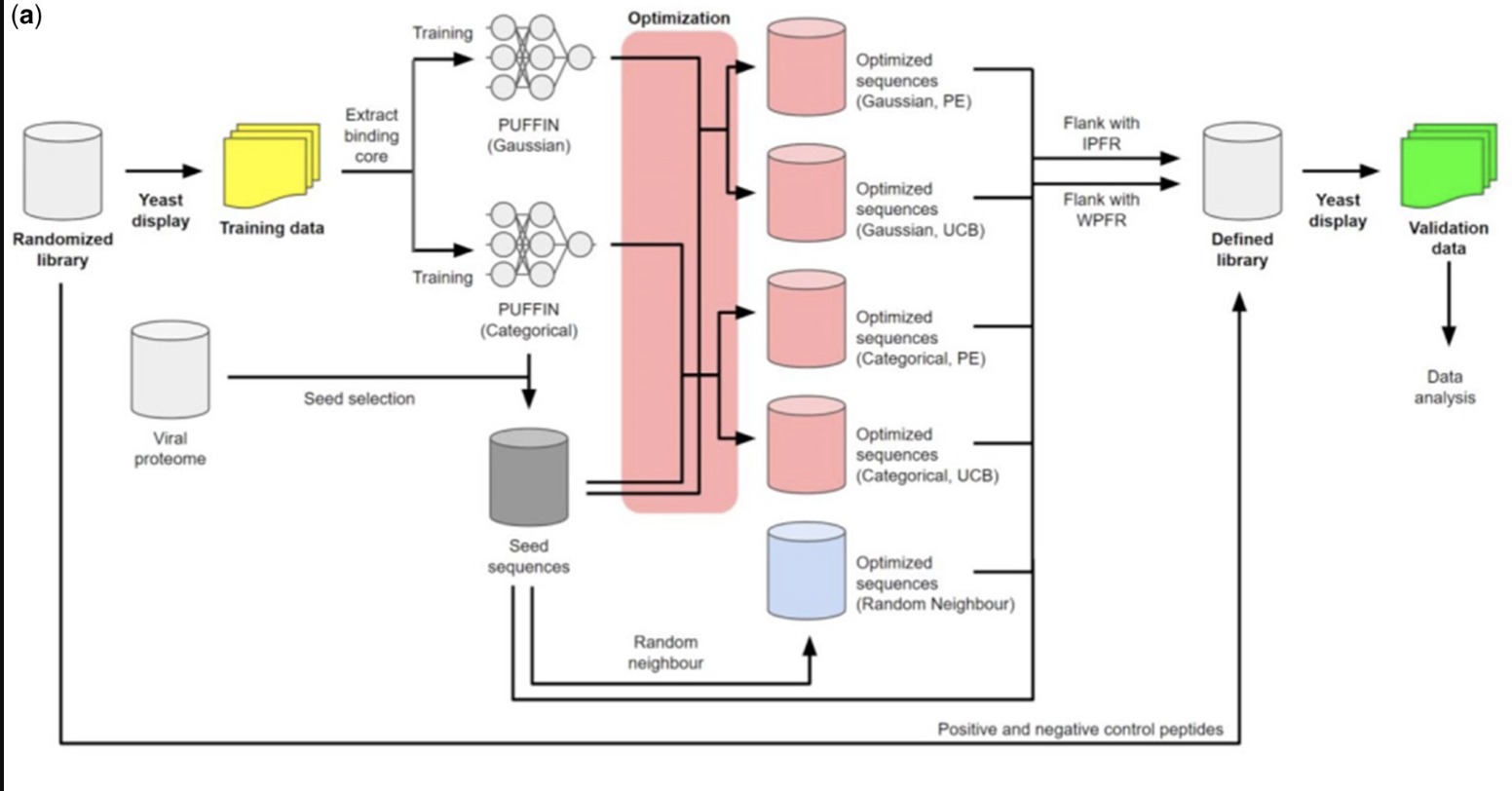
Zheng Dai, Brooke D Huisman, Haoyang Zeng, Brandon Carter, Siddhartha Jain, Michael E Birnbaum, David K Gifford
Bioinformatics. Volume 37, Issue 19
DOI: 10.1093/bioinformatics/btab131
General and cell-type-specific aspects of the motor neuron maturation transcriptional program
Building a nervous system is a protracted process that starts with the specification of individual neuron types and ends with the formation of mature neural circuits. The molecular mechanisms that regulate the temporal progression of maturation in individual cell types remain poorly understood. In this work, we have mapped the gene expression and chromatin accessibility changes in mouse spinal motor neurons throughout their lifetimes. We found that both motor neuron gene expression and putative regulatory elements are dynamic during the first three weeks of postnatal life, when motor circuits are maturing. Genes that are up-regulated during this time contribute to adult motor neuron diversity and function. Almost all of the chromatin regions that gain accessibility during maturation are motor neuron specific, yet a majority of the transcription factor binding motifs enriched in these regions are shared with other mature neurons. Collectively, these findings suggest that a core transcriptional program operates in a context-dependent manner to access cell-type-specific cis-regulatory systems associated with maturation genes. Discovery of general principles governing neuronal maturation might inform methods for transcriptional reprogramming of neuronal age and for improved modelling of age-related neurodegenerative diseases.
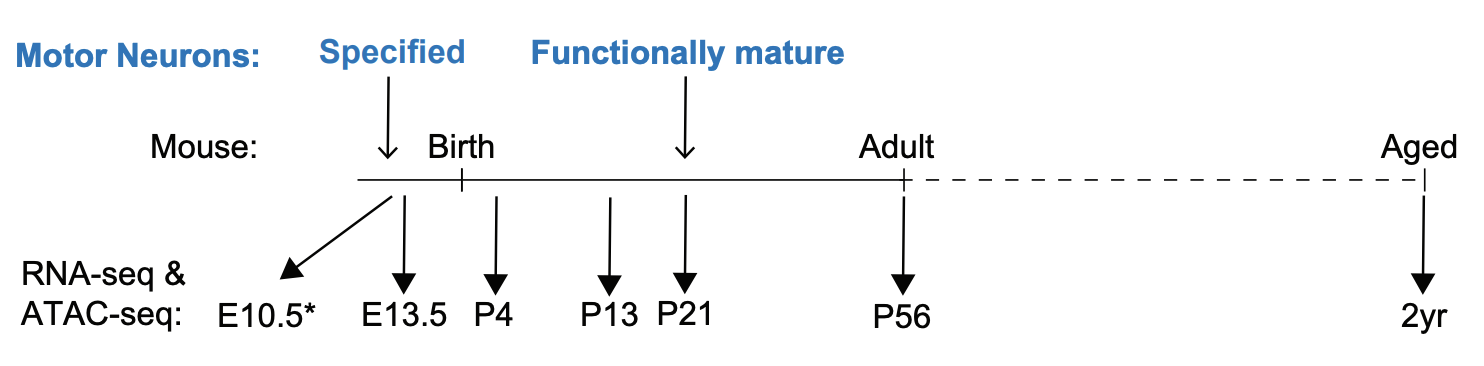
Tulsi Patel, Jennifer Hammelman, Michael Closser, David K. Gifford, Hynek Wichterle
bioRxiv.
DOI: 10.1101/2021.03.05.434185
Machine learning based CRISPR gRNA design for therapeutic exon skipping
Restoring gene function by the induced skipping of deleterious exons has been shown to be effective for treating genetic disorders. However, many of the clinically successful therapies for exon skipping are transient oligonucleotide-based treatments that require frequent dosing. CRISPR-Cas9 based genome editing that causes exon skipping is a promising therapeutic modality that may offer permanent alleviation of genetic disease. We show that machine learning can select Cas9 guide RNAs that disrupt splice acceptors and cause the skipping of targeted exons. We experimentally measured the exon skipping frequencies of a diverse genome-integrated library of 791 splice sequences targeted by 1,063 guide RNAs in mouse embryonic stem cells. We found that our method, SkipGuide, is able to identify effective guide RNAs with a precision of 0.68 (50% threshold predicted exon skipping frequency) and 0.93 (70% threshold predicted exon skipping frequency). We anticipate that SkipGuide will be useful for selecting guide RNA candidates for evaluation of CRISPR-Cas9-mediated exon skipping therapy.
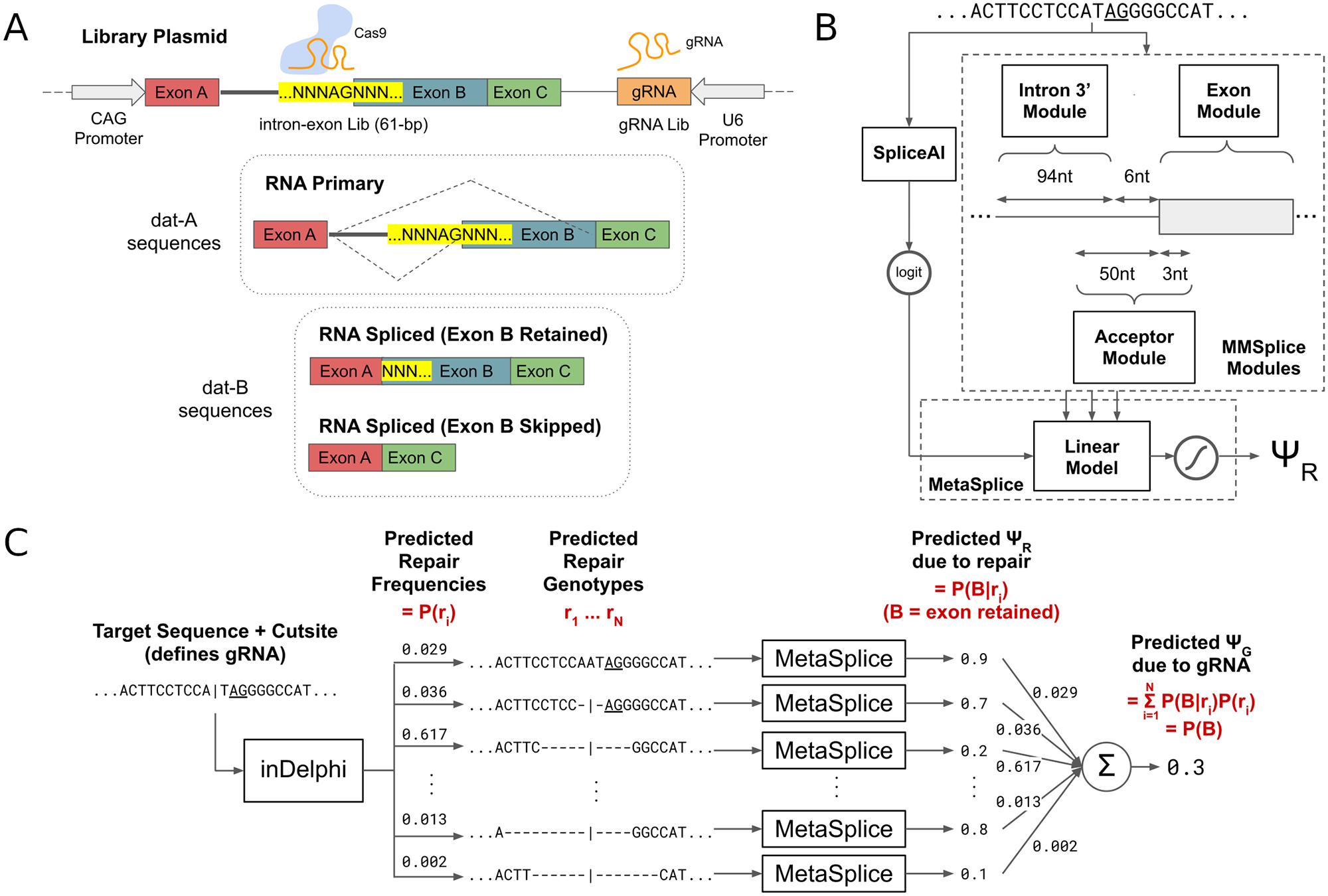
Wilson Louie, Max W. Shen, Zakir Tahiry, Sophia Zhang, Daniel Worstell, Christopher A. Cassa, Richard I. Sherwood, David K. Gifford
PLoS Computational Biology. Volume 17, Issue 1, e1008605
DOI: 10.1371/journal.pcbi.1008605
2020
Predicted Cellular Immunity Population Coverage Gaps for SARS-CoV-2 Subunit Vaccines and Their Augmentation by Compact Peptide Sets
Subunit vaccines induce immunity to a pathogen by presenting a component of the pathogen and thus inherently limit the representation of pathogen peptides for cellular immunity-based memory. We find that severe acute respiratory syndrome coronavirus 2 (SARS-CoV-2) subunit peptides may not be robustly displayed by the major histocompatibility complex (MHC) molecules in certain individuals. We introduce an augmentation strategy for subunit vaccines that adds a small number of SARS-CoV-2 peptides to a vaccine to improve the population coverage of pathogen peptide display. Our population coverage estimates integrate clinical data on peptide immunogenicity in convalescent COVID-19 patients and machine learning predictions. We evaluate the population coverage of 9 different subunits of SARS-CoV-2, including 5 functional domains and 4 full proteins, and augment each of them to fill a predicted coverage gap.
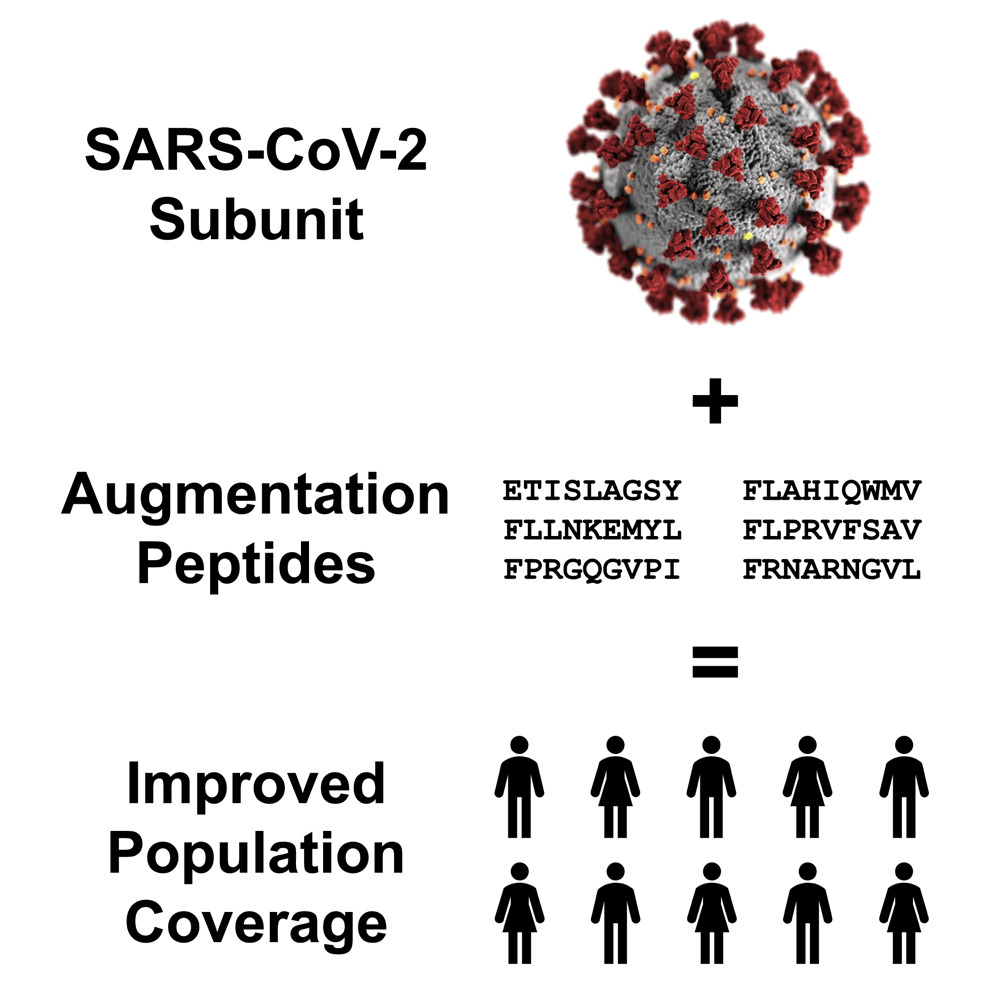
Ge Liu, Brandon Carter, and David K. Gifford
Cell Systems. Volume 12, P1–6, February 17, 2021
DOI: 10.1016/j.cels.2020.11.010
Comprehensive Mapping of Key Regulatory Networks that Drive Oncogene Expression
Gene expression is controlled by the collective binding of transcription factors to cis-regulatory regions. Deciphering gene-centered regulatory networks is vital to understanding and controlling gene misexpression in human disease; however, systematic approaches to uncovering regulatory networks have been lacking. Here we present high-throughput interrogation of gene-centered activation networks (HIGAN), a pipeline that employs a suite of multifaceted genomic approaches to connect upstream signaling inputs, trans-acting TFs, and cis-regulatory elements. We apply HIGAN to understand the aberrant activation of the cytidine deaminase APOBEC3B, an intrinsic source of cancer hypermutation. We reveal that nuclear factor κB (NF-κB) and AP-1 pathways are the most salient trans-acting inputs, with minor roles for other inflammatory pathways. We identify a cis-regulatory architecture dominated by a major intronic enhancer that requires coordinated NF-κB and AP-1 activity with secondary inputs from distal regulatory regions. Our data demonstrate how integration of cis and trans genomic screening platforms provides a paradigm for building gene-centered regulatory networks.
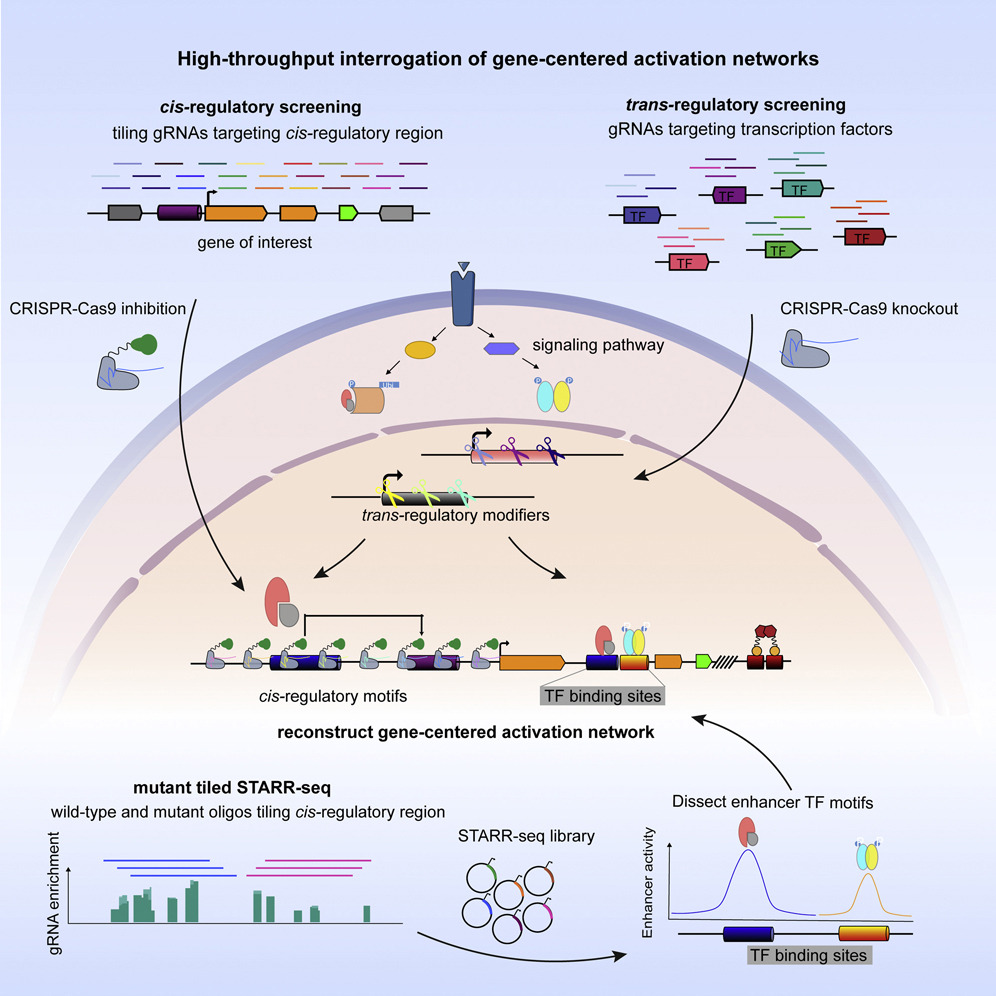
Lin Lin, Benjamin Holmes, Max W. Shen, Darnell Kammeron, Niels Geijsen, David K. Gifford, and Richard I. Sherwood
Cell Reports. Volume 33, Issue 8, 24 November 2020, 108426
DOI: 10.1016/j.celrep.2020.108426
Extracting and Interpreting the Effects of Higher Order Sequence Features on Peptide MHC Binding
Understanding the factors contributing to peptide MHC (pMHC) affinity is critical for the study of immune responses and the development of novel therapeutics. Developments in yeast display platforms have enabled the collection of pMHC binding data for vast libraries of peptides. However, methods for interpreting this data are still at an early stage. In this work we propose an approach for extracting peptide sequence features that affect pMHC binding from such datasets. In the process we develop the theoretical framework for fitting and interpreting these features. We demonstrate that these features accurately capture the kinetics underlying pMHC binding, and can be used to predict pMHC binding well enough to rival the current state of the art. We then analyze the extracted factors and show that they correlate with our current structural understanding of MHC molecules. Finally, we discuss the implication these factors have on the complexity of peptide engineering.
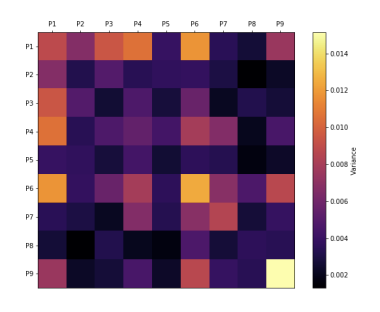
Zheng Dai, Brooke D Huisman, Michael E Birnbaum, David K Gifford
bioRxiv.
DOI: 10.1101/2020.11.20.392233
Chemogenetic System Demonstrates That Cas9 Longevity Impacts Genome Editing Outcomes
Prolonged Cas9 activity can hinder genome engineering as it causes off-target effects, genotoxicity, heterogeneous genome-editing outcomes, immunogenicity, and mosaicism in embryonic editing—issues which could be addressed by controlling the longevity of Cas9. Though some temporal controls of Cas9 activity have been developed, only cumbersome systems exist for modifying the lifetime. Here, we have developed a chemogenetic system that brings Cas9 in proximity to a ubiquitin ligase, enabling rapid ubiquitination and degradation of Cas9 by the proteasome. Despite the large size of Cas9, we were able to demonstrate efficient degradation in cells from multiple species. Furthermore, by controlling the Cas9 lifetime, we were able to bias the DNA repair pathways and the genotypic outcome for both templated and nontemplated genome editing. Finally, we were able to dosably control the Cas9 activity and specificity to ameliorate the off-target effects. The ability of this system to change the Cas9 lifetime and, therefore, bias repair pathways and specificity in the desired direction allows precision control of the genome editing outcome.
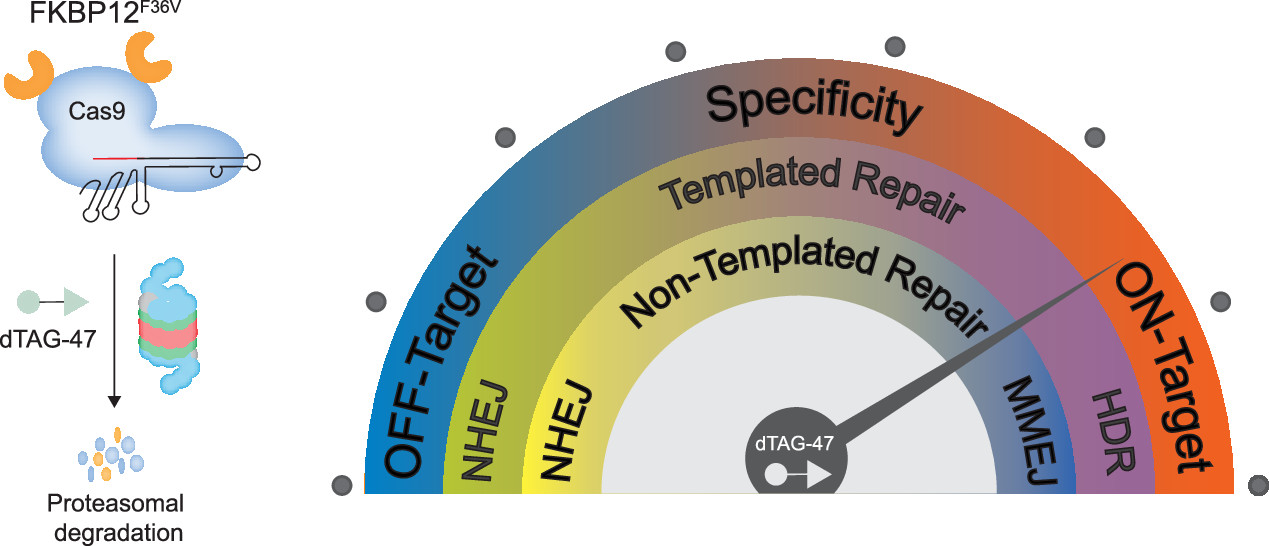
Vedagopuram Sreekanth, Qingxuan Zhou, Praveen Kokkonda, Heysol C. Bermudez-Cabrera, Donghyun Lim, Benjamin K. Law, Benjamin R. Holmes, Santosh K. Chaudhary, Rajaiah Pergu, Brittany S. Leger, James A. Walker, David K. Gifford, Richard I. Sherwood, and Amit Choudhary
ACS Cent. Sci.. 2020
DOI: 10.1021/acscentsci.0c00129
Identification of determinants of differential chromatin accessibility through a massively parallel genome-integrated reporter assay
A key mechanism in cellular regulation is the ability of the transcriptional machinery to physically access DNA. Transcription factors interact with DNA to alter the accessibility of chromatin, which enables changes to gene expression during development or disease or as a response to environmental stimuli. However, the regulation of DNA accessibility via the recruitment of transcription factors is difficult to study in the context of the native genome because every genomic site is distinct in multiple ways. Here we introduce the multiplexed integrated accessibility assay (MIAA), an assay that measures chromatin accessibility of synthetic oligonucleotide sequence libraries integrated into a controlled genomic context with low native accessibility. We apply MIAA to measure the effects of sequence motifs on cell type–specific accessibility between mouse embryonic stem cells and embryonic stem cell–derived definitive endoderm cells, screening 7905 distinct DNA sequences. MIAA recapitulates differential accessibility patterns of 100-nt sequences derived from natively differential genomic regions, identifying E-box motifs common to epithelial–mesenchymal transition driver transcription factors in stem cell–specific accessible regions that become repressed in endoderm. We show that a single binding motif for a key regulatory transcription factor is sufficient to open chromatin, and classify sets of stem cell–specific, endoderm-specific, and shared accessibility-modifying transcription factor motifs. We also show that overexpression of two definitive endoderm transcription factors, T and Foxa2, results in changes to accessibility in DNA sequences containing their respective DNA-binding motifs and identify preferential motif arrangements that influence accessibility.
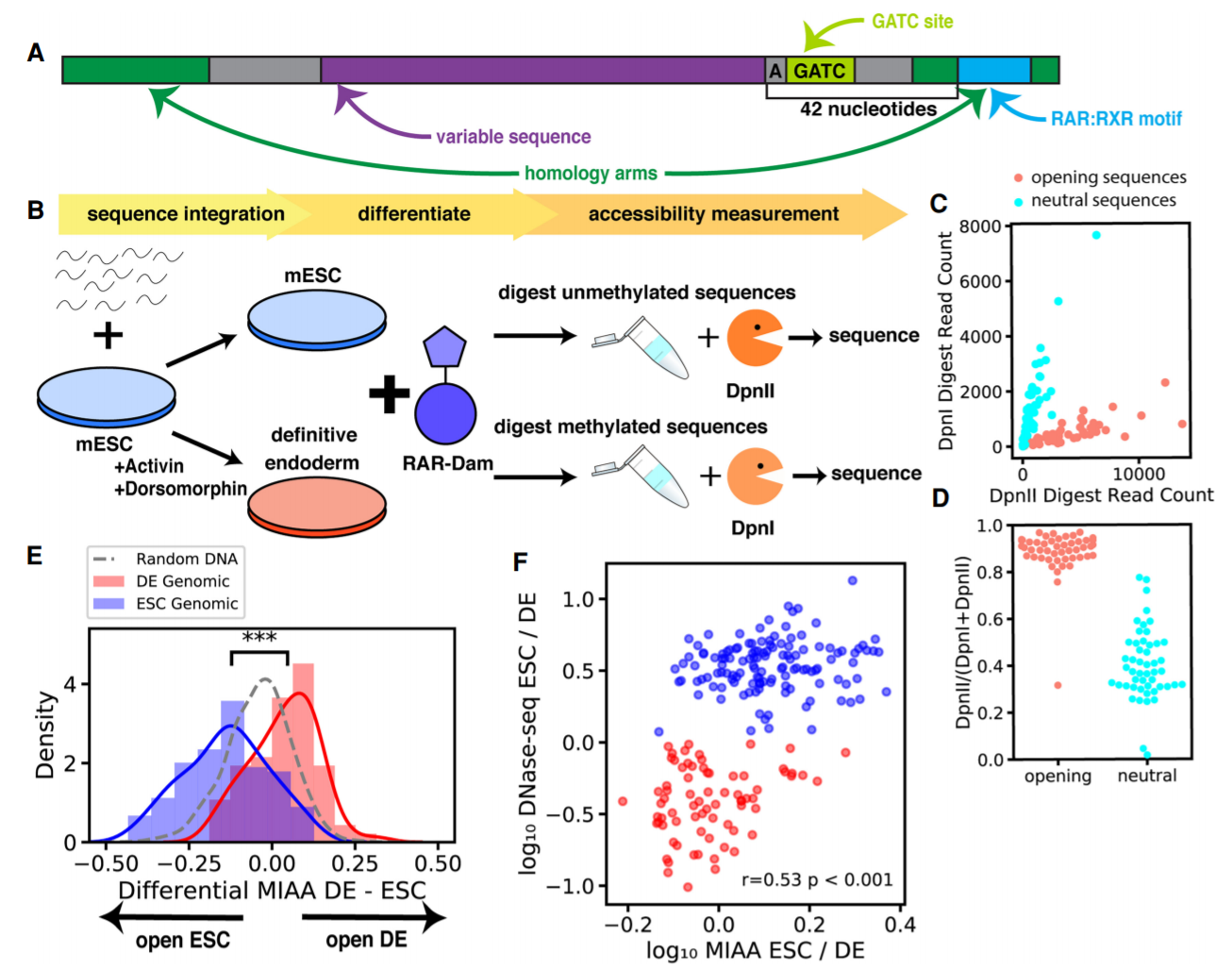
Jennifer Hammelman, Konstantin Krismer, Budhaditya Banerjee, David K. Gifford and Richard I. Sherwood
Genome Research. 2020
DOI: 10.1101/gr.263228.120
Computationally Optimized SARS-CoV-2 MHC Class I and II Vaccine Formulations Predicted to Target Human Haplotype Distributions
We present a combinatorial machine learning method to evaluate and optimize peptide vaccine formulations for SARS-CoV-2. Our approach optimizes the presentation likelihood of a diverse set of vaccine peptides conditioned on a target human-population HLA haplotype distribution and expected epitope drift. Our proposed SARS-CoV-2 MHC class I vaccine formulations provide 93.21% predicted population coverage with at least five vaccine peptide-HLA average hits per person (≥ 1 peptide: 99.91%) with all vaccine peptides perfectly conserved across 4,690 geographically sampled SARS-CoV-2 genomes. Our proposed MHC class II vaccine formulations provide 97.21% predicted coverage with at least five vaccine peptide-HLA average hits per person with all peptides having an observed mutation probability of ≤ 0.001. We provide an open-source implementation of our design methods (OptiVax), vaccine evaluation tool (EvalVax), as well as the data used in our design efforts here: https://github.com/gifford-lab/optivax.
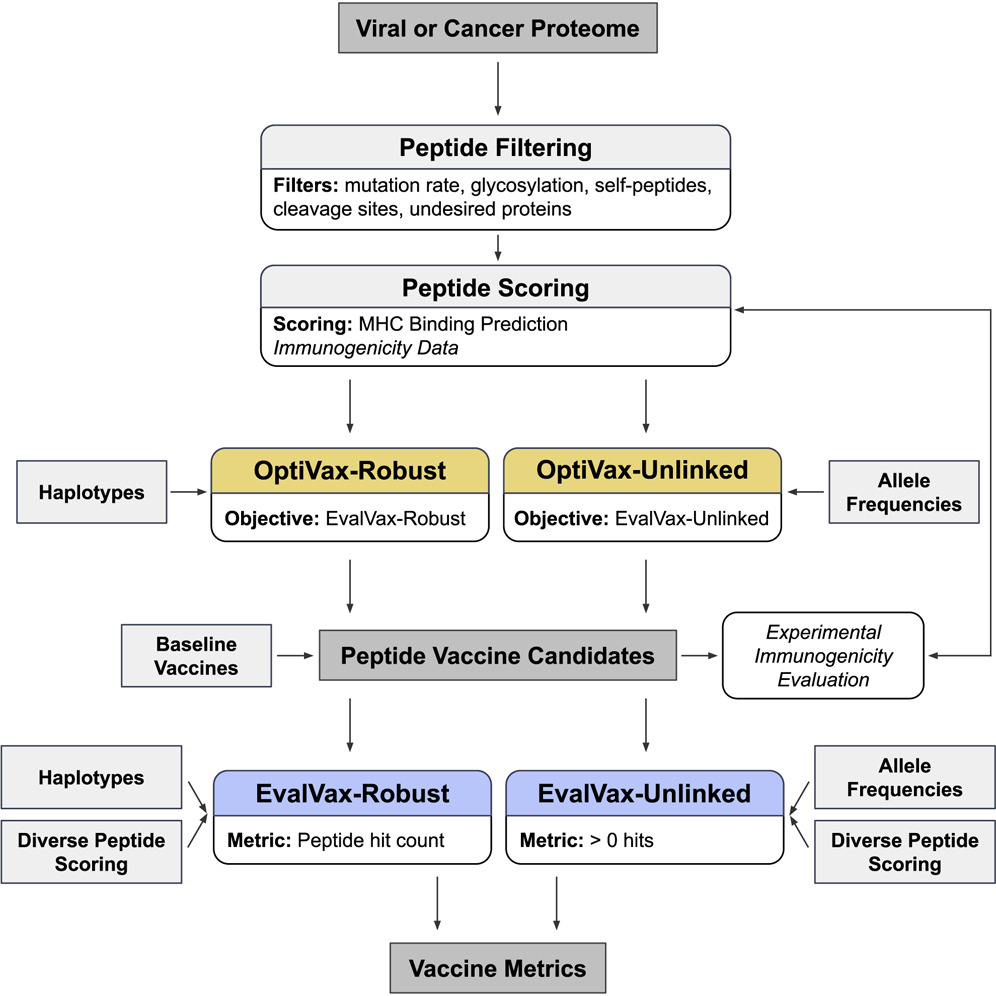
Ge Liu, Brandon Carter, Trenton Bricken, Siddhartha Jain, Mathias Viard, Mary Carrington, and David K. Gifford
Cell Systems. Volume 11, Issue 2, P131-144.E6, August 26, 2020
DOI: 10.1016/j.cels.2020.06.009
Expanded encyclopaedias of DNA elements in the human and mouse genomes
The human and mouse genomes contain instructions that specify RNAs and proteins and govern the timing, magnitude, and cellular context of their production. To better delineate these elements, phase III of the Encyclopedia of DNA Elements (ENCODE) Project has expanded analysis of the cell and tissue repertoires of RNA transcription, chromatin structure and modification, DNA methylation, chromatin looping, and occupancy by transcription factors and RNA-binding proteins. Here we summarize these efforts, which have produced 5,992 new experimental datasets, including systematic determinations across mouse fetal development. All data are available through the ENCODE data portal (https://www.encodeproject.org), including phase II ENCODE1 and Roadmap Epigenomics2 data. We have developed a registry of 926,535 human and 339,815 mouse candidate cis-regulatory elements, covering 7.9 and 3.4% of their respective genomes, by integrating selected datatypes associated with gene regulation, and constructed a web-based server (SCREEN; http://screen.encodeproject.org) to provide flexible, user-defined access to this resource. Collectively, the ENCODE data and registry provide an expansive resource for the scientific community to build a better understanding of the organization and function of the human and mouse genomes.
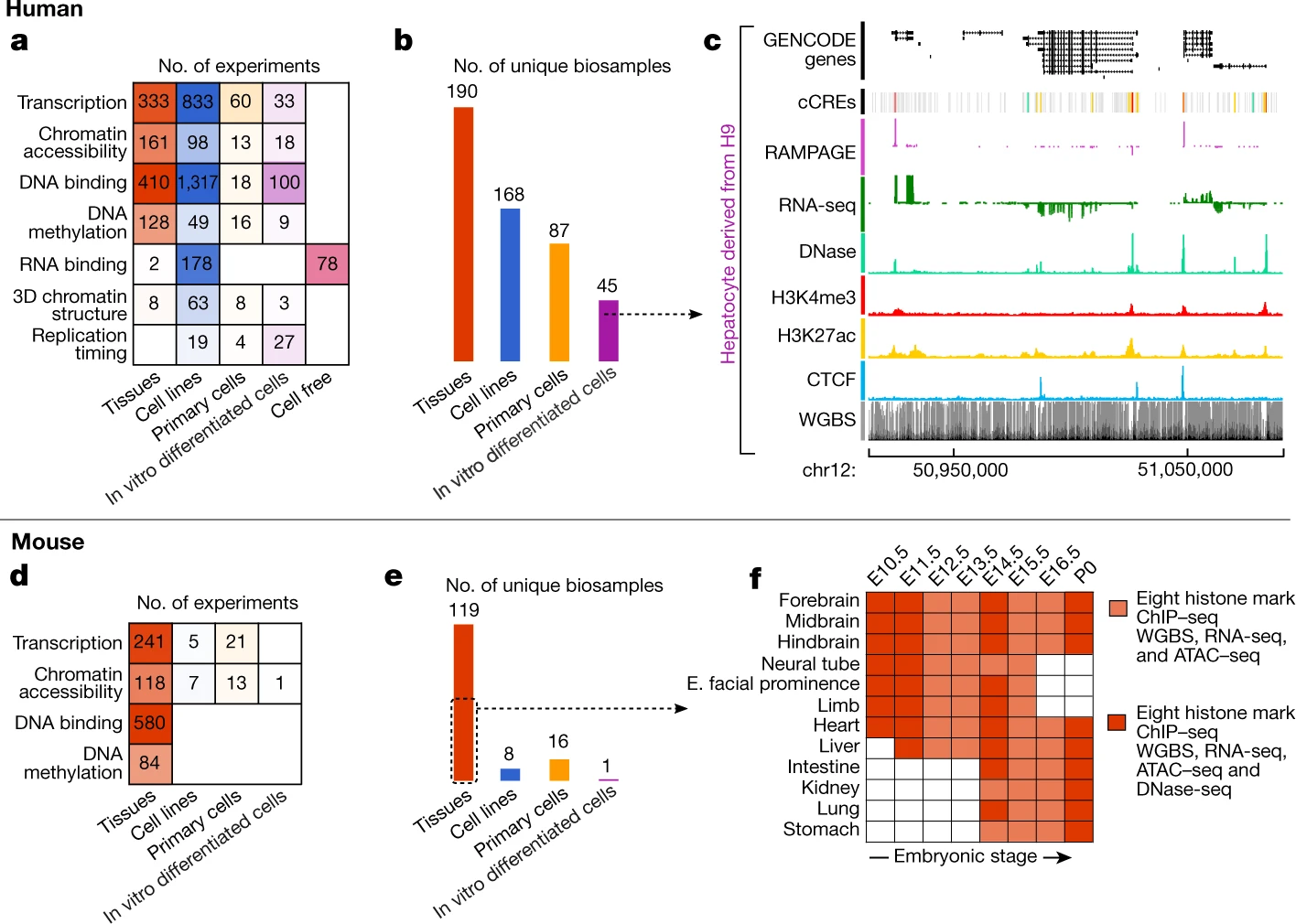
The ENCODE Project Consortium, Jill E. Moore, Michael J. Purcaro, Henry E. Pratt, Charles B. Epstein, Noam Shoresh, Jessika Adrian, Trupti Kawli, Carrie A. Davis, Alexander Dobin, Rajinder Kaul, Jessica Halow, Eric L. Van Nostrand, Peter Freese, David U. Gorkin, Yin Shen, Yupeng He, Mark Mackiewicz, Florencia Pauli-Behn, Brian A. Williams, Ali Mortazavi, Cheryl A. Keller, Xiao-Ou Zhang, Shaimae I. Elhajjajy, Jack Huey, Diane E. Dickel, Valentina Snetkova, Xintao Wei, Xiaofeng Wang, Juan Carlos Rivera-Mulia, Joel Rozowsky, Jing Zhang, Surya B. Chhetri, Jialing Zhang, Alec Victorsen, Kevin P. White, Axel Visel, Gene W. Yeo, Christopher B. Burge, Eric Lécuyer, David M. Gilbert, Job Dekker, John Rinn, Eric M. Mendenhall, Joseph R. Ecker, Manolis Kellis, Robert J. Klein, William S. Noble, Anshul Kundaje, Roderic Guigó, Peggy J. Farnham, J. Michael Cherry, Richard M. Myers, Bing Ren, Brenton R. Graveley, Mark B. Gerstein, Len A. Pennacchio, Michael P. Snyder, Bradley E. Bernstein, Barbara Wold, Ross C. Hardison, Thomas R. Gingeras, John A. Stamatoyannopoulos, and Zhiping Weng
Nature. 583, pages699–710(2020)
DOI: 10.1038/s41586-020-2493-4
Perspectives on ENCODE
The Encylopedia of DNA Elements (ENCODE) Project launched in 2003 with the long-term goal of developing a comprehensive map of functional elements in the human genome. These included genes, biochemical regions associated with gene regulation (for example, transcription factor binding sites, open chromatin, and histone marks) and transcript isoforms. The marks serve as sites for candidate cis-regulatory elements (cCREs) that may serve functional roles in regulating gene expression. The project has been extended to model organisms, particularly the mouse. In the third phase of ENCODE, nearly a million and more than 300,000 cCRE annotations have been generated for human and mouse, respectively, and these have provided a valuable resource for the scientific community.
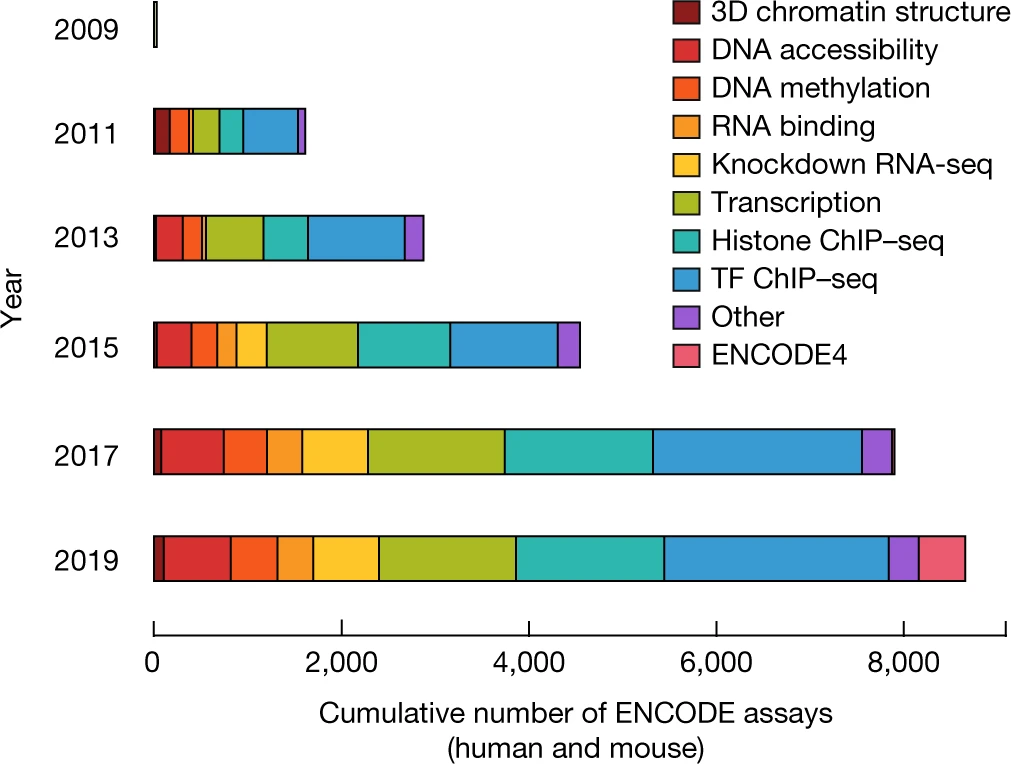
The ENCODE Project Consortium, Michael P. Snyder, Thomas R. Gingeras, Jill E. Moore, Zhiping Weng, Mark B. Gerstein, Bing Ren, Ross C. Hardison, John A. Stamatoyannopoulos, Brenton R. Graveley, Elise A. Feingold, Michael J. Pazin, Michael Pagan, Daniel A. Gilchrist, Benjamin C. Hitz, J. Michael Cherry, Bradley E. Bernstein, Eric M. Mendenhall, Daniel R. Zerbino, Adam Frankish, Paul Flicek, and Richard M. Myers
Nature. 583, pages693–698(2020)
DOI: 10.1038/s41586-020-2449-8
A Multiplexed Barcodelet Single-Cell RNA-Seq Approach Elucidates Combinatorial Signaling Pathways that Drive ESC Differentiation
Empirical optimization of stem cell differentiation protocols is time consuming, is laborintensive, and typically does not comprehensively interrogate all relevant signaling pathways. Here we describe barcodelet single-cell RNA sequencing (barRNA-seq), which enables systematic exploration of cellular perturbations by tagging individual cells with RNA “barcodelets” to identify them on the basis of the treatments they receive. We apply barRNA-seq to simultaneously manipulate up to seven developmental pathways and study effects on embryonic stem cell (ESC) germ layer specification and mesodermal specification, uncovering combinatorial effects of signaling pathway activation on gene expression. We further develop a data-driven framework for identifying combinatorial signaling perturbations that drive cells toward specific fates, including several annotated in an existing scRNA-seq gastrulation atlas, and use this approach to guide ESC differentiation into a notochord-like population. We expect that barRNA-seq will have broad utility for investigating and understanding how cooperative signaling pathways drive cell fate acquisition.
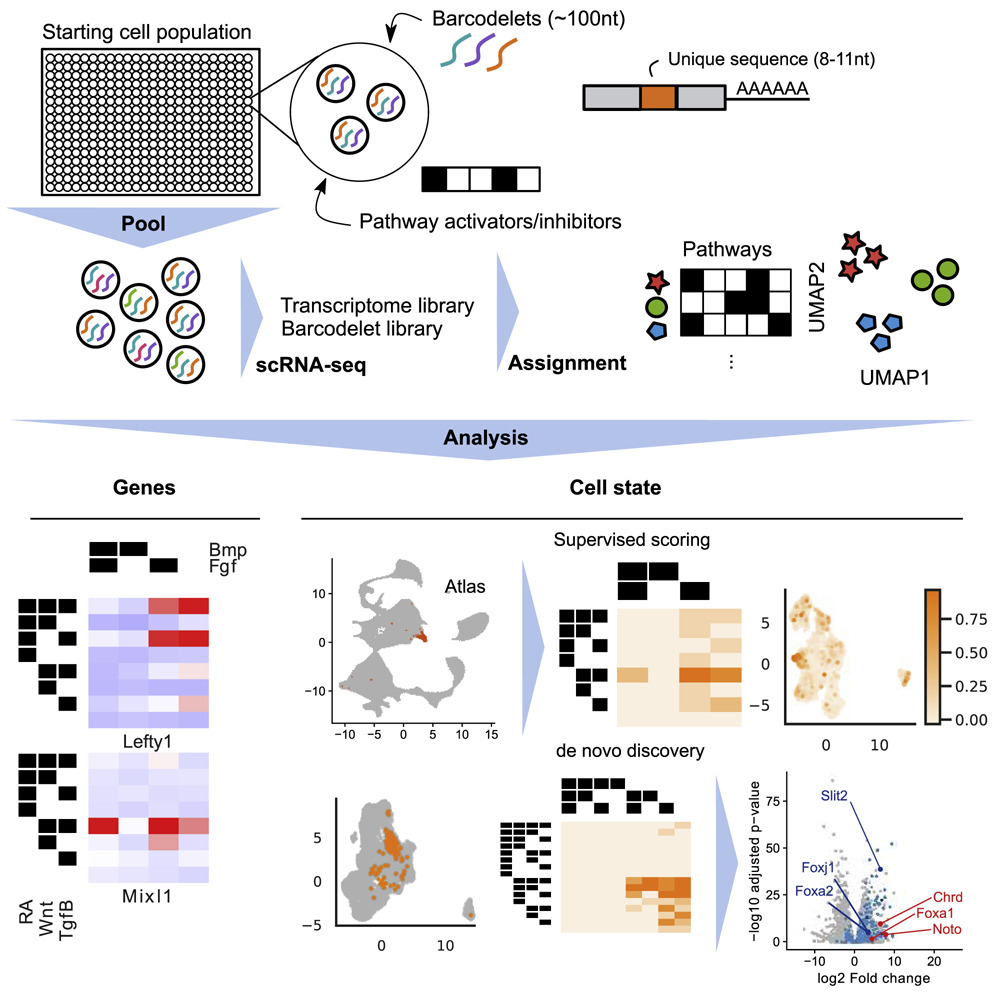
Grace Hui Ting Yeo, Lin Lin, Celine Yueyue Qi, Minsun Cha, David K. Gifford, and Richard I. Sherwood
Cell Stem Cell. Volume 26, Issue 6, 4 June 2020, Pages 938-950.e6
DOI: 10.1016/j.stem.2020.04.020
IDR2D identifies reproducible genomic interactions
Chromatin interaction data from protocols such as ChIA-PET, HiChIP, and HiC provide valuable insights into genome organization and gene regulation, but can include spurious interactions that do not reflect underlying genome biology. We introduce a generalization of the Irreproducible Discovery Rate (IDR) method called IDR2D that identifies replicable interactions shared by chromatin interaction experiments. IDR2D provides a principled set of interactions and eliminates artifacts from single experiments. The method is available as a Bioconductor package for the R community, as well as an online service at https://idr2d.mit.edu.
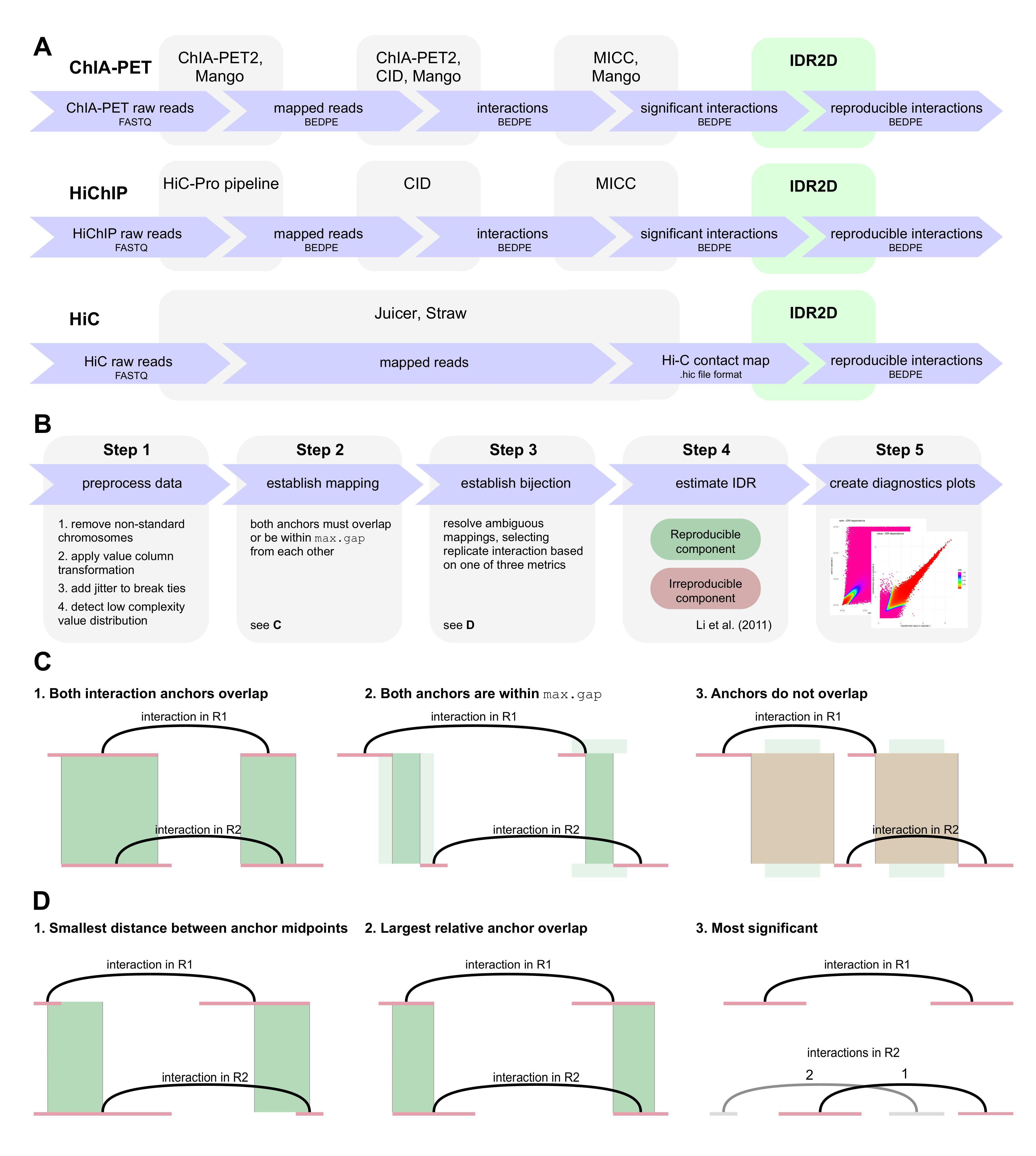
Konstantin Krismer, Yuchun Guo, and David K. Gifford
Nucleic Acids Research. Volume 48, Issue 6, 06 April 2020, Page e31
DOI: 10.1093/nar/gkaa030
2019
Antibody Complementarity Determining Region Design Using High-Capacity Machine Learning
The precise targeting of antibodies and other protein therapeutics is required for their proper function and the elimination of deleterious off-target effects. Often the molecular structure of a therapeutic target is unknown and randomized methods are used to design antibodies without a model that relates antibody sequence to desired properties. Here we present a machine learning method that can design human Immunoglobulin G (IgG) antibodies with target affinities that are superior to candidates from phage display panning experiments within a limited design budget. We also demonstrate that machine learning can improve target-specificity by the modular composition of models from different experimental campaigns, enabling a new integrative approach to improving target specificity. Our results suggest a new path for the discovery of therapeutic molecules by demonstrating that predictive and differentiable models of antibody binding can be learned from high-throughput experimental data without the need for target structural data.
Significance Antibody based therapeutics must meet both affinity and specificity metrics, and existing in vitro methods for meeting these metrics are based upon randomization and empirical testing. We demonstrate that with sufficient target-specific training data machine learning can suggest novel antibody variable domain sequences that are superior to those observed during training. Our machine learning method does not require any target structural information. We further show that data from disparate antibody campaigns can be combined by machine learning to improve antibody specificity.
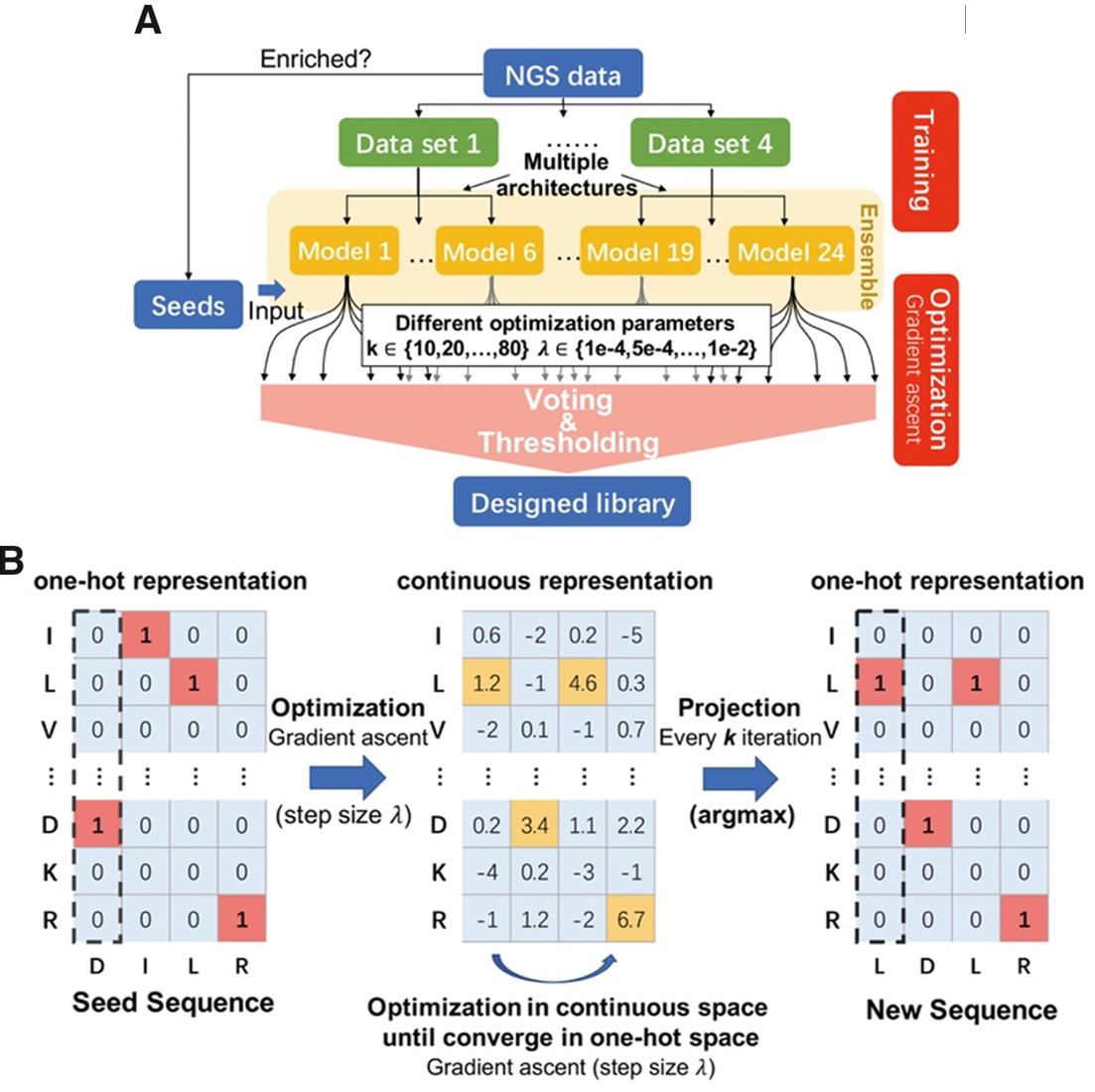
Ge Liu, Haoyang Zeng, Jonas Mueller, Brandon Carter, Ziheng Wang, Jonas Schilz, Geraldine Horny, Michael E. Birnbaum, Stefan Ewert, and David K. Gifford
Bioinformatics. btz895
DOI: 10.1093/bioinformatics/btz895
Quantification of Uncertainty in Peptide-MHC Binding Prediction Improves High-Affinity Peptide Selection for Therapeutic Design
The computational identification of peptides that can bind the major histocompatibility complex (MHC) with high affinity is an essential step in developing personal immunotherapies and vaccines. We introduce PUFFIN, a deep residual network-based computational approach that quantifies uncertainty in peptide-MHC affinity prediction that arises from observational noise and the lack of relevant training examples. With PUFFIN’s uncertainty metrics, we define binding likelihood, the probability a peptide binds to a given MHC allele at a specified affinity threshold. Compared to affinity point estimates, we find that binding likelihood correlates better with the observed affinity and reduces false positives in high-affinity peptide design. When applied to examine an existing peptide vaccine, PUFFIN identifies an alternative vaccine formulation with higher binding likelihood. PUFFIN is freely available for download at https://github.com/gifford-lab/PUFFIN.
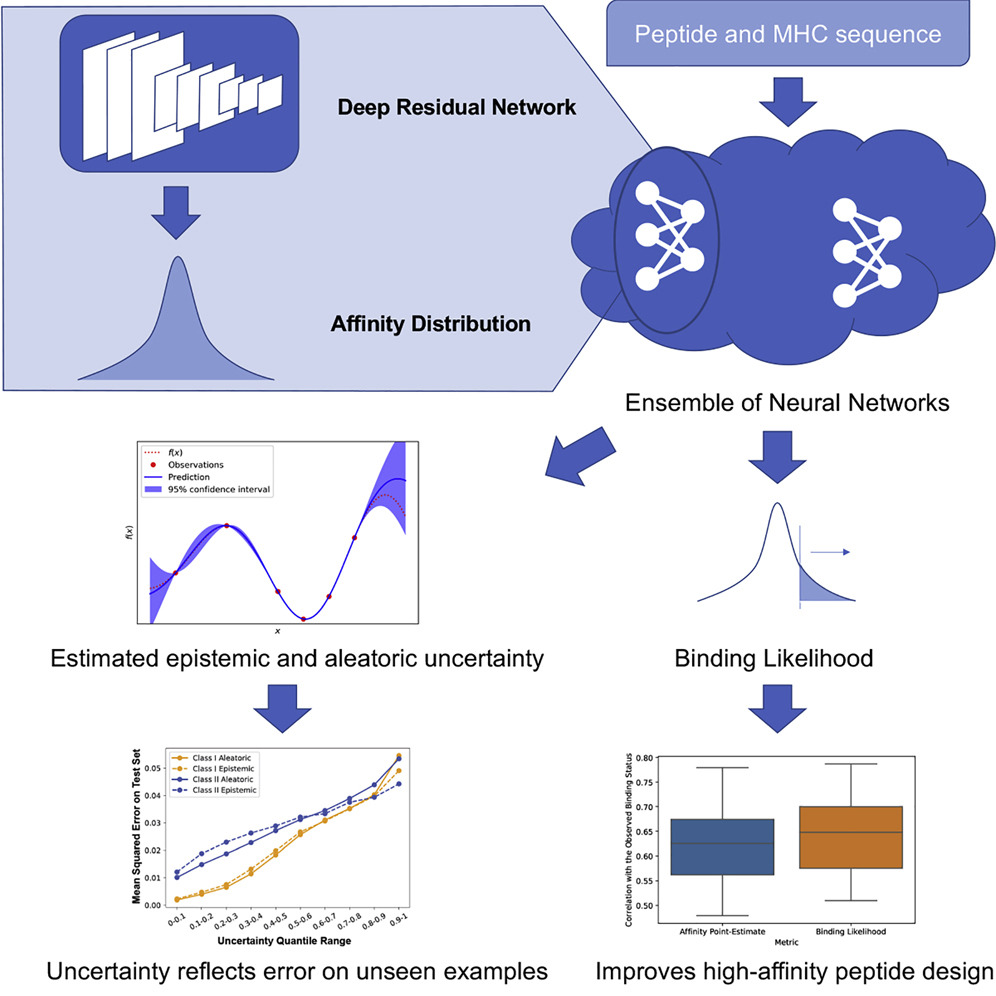
Haoyang Zeng and David K. Gifford
Cell Systems. Volume 9, Issue 2, 28 August 2019, Pages 159-166.e3
DOI: 10.1016/j.cels.2019.05.004
Visualizing complex feature interactions and feature sharing in genomic deep neural networks
Background
Visualization tools for deep learning models typically focus on discovering key input features without considering how such low level features are combined in intermediate layers to make decisions. Moreover, many of these methods examine a network’s response to specific input examples that may be insufficient to reveal the complexity of model decision making.
Results
We present DeepResolve, an analysis framework for deep convolutional models of genome function that visualizes how input features contribute individually and combinatorially to network decisions. Unlike other methods, DeepResolve does not depend upon the analysis of a predefined set of inputs. Rather, it uses gradient ascent to stochastically explore intermediate feature maps to 1) discover important features, 2) visualize their contribution and interaction patterns, and 3) analyze feature sharing across tasks that suggests shared biological mechanism. We demonstrate the visualization of decision making using our proposed method on deep neural networks trained on both experimental and synthetic data. DeepResolve is competitive with existing visualization tools in discovering key sequence features, and identifies certain negative features and non-additive feature interactions that are not easily observed with existing tools. It also recovers similarities between poorly correlated classes which are not observed by traditional methods. DeepResolve reveals that DeepSEA’s learned decision structure is shared across genome annotations including histone marks, DNase hypersensitivity, and transcription factor binding. We identify groups of TFs that suggest known shared biological mechanism, and recover correlation between DNA hypersensitivities and TF/Chromatin marks.
Conclusions
DeepResolve is capable of visualizing complex feature contribution patterns and feature interactions that contribute to decision making in genomic deep convolutional networks. It also recovers feature sharing and class similarities which suggest interesting biological mechanisms. DeepResolve is compatible with existing visualization tools and provides complementary insights.
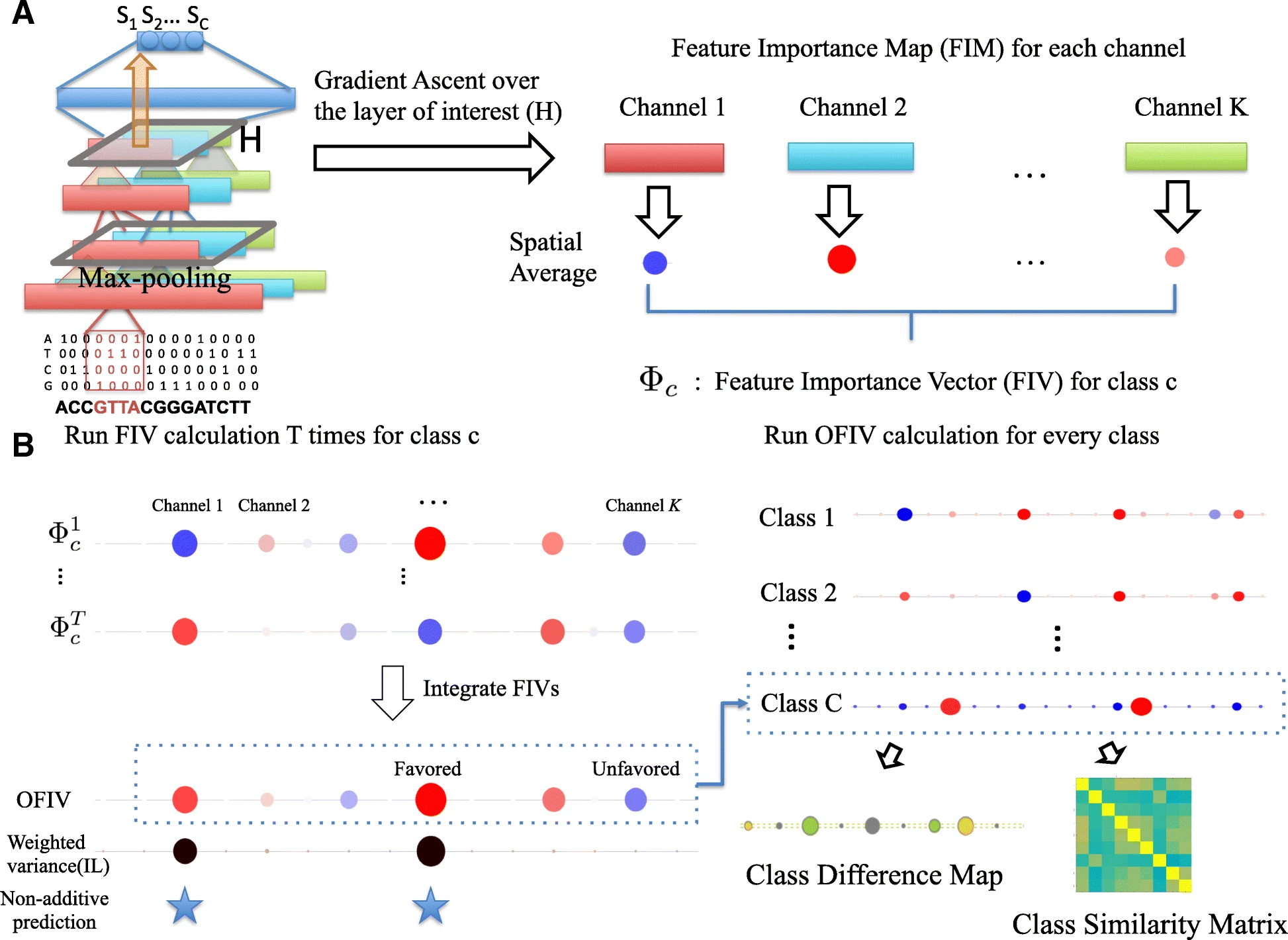
Ge Liu, Haoyang Zeng, and David K. Gifford
BMC Bioinformatics. 20, 401 (2019)
DOI: 10.1186/s12859-019-2957-4
DeepLigand: accurate prediction of MHC class I ligands using peptide embedding
Motivation
The computational modeling of peptide display by class I major histocompatibility complexes (MHCs) is essential for peptide-based therapeutics design. Existing computational methods for peptide-display focus on modeling the peptide-MHC-binding affinity. However, such models are not able to characterize the sequence features for the other cellular processes in the peptide display pathway that determines MHC ligand selection.
Results
We introduce a semi-supervised model, DeepLigand that outperforms the state-of-the-art models in MHC Class I ligand prediction. DeepLigand combines a peptide language model and peptide binding affinity prediction to score MHC class I peptide presentation. The peptide language model characterizes sequence features that correspond to secondary factors in MHC ligand selection other than binding affinity. The peptide embedding is learned by pre-training on natural ligands, and can discriminate between ligands and non-ligands in the absence of binding affinity prediction. Although conventional affinity-based models fail to classify peptides with moderate affinities, DeepLigand discriminates ligands from non-ligands with consistently high accuracy.
Availability and implementation
We make DeepLigand available at https://github.com/gifford-lab/DeepLigand.
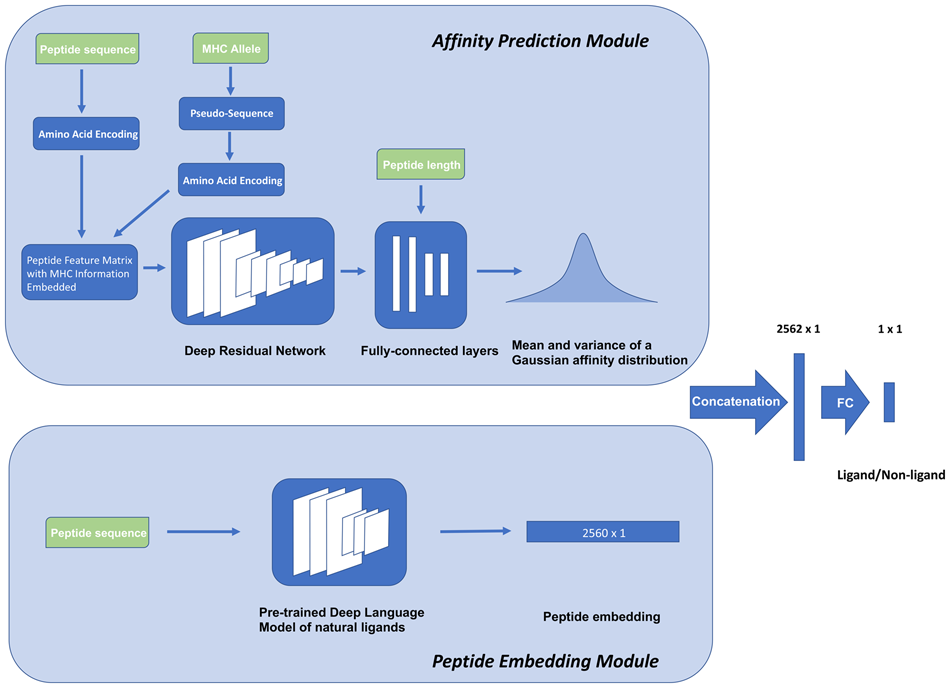
Haoyang Zeng and David K Gifford
Bioinformatics. Volume 35, Issue 14, July 2019, Pages i278–i283
DOI: 10.1093/bioinformatics/btz330
Maximizing Overall Diversity for Improved Uncertainty Estimates in Deep Ensembles
The inaccuracy of neural network models on inputs that do not stem from the training data distribution is both problematic and at times unrecognized. Model uncertainty estimation can address this issue, where uncertainty estimates are often based on the variation in predictions produced by a diverse ensemble of models applied to the same input. Here we describe Maximize Overall Diversity (MOD), a straightforward approach to improve ensemble-based uncertainty estimates by encouraging larger overall diversity in ensemble predictions across all possible inputs that might be encountered in the future. When applied to various neural network ensembles, MOD significantly improves predictive performance for out-of-distribution test examples without sacrificing in-distribution performance on 38 Protein-DNA binding regression datasets, 9 UCI datasets, and the IMDB-Wiki image dataset. Across many Bayesian optimization tasks, the performance of UCB acquisition is also greatly improved by leveraging MOD uncertainty estimates.
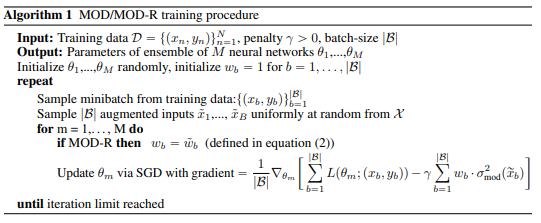
Siddhartha Jain, Ge Liu, Jonas Mueller, and David Gifford
arXiv. preprint arXiv:1906.07380
arXiv: 1906.07380
Wnt Signaling Separates the Progenitor and Endocrine Compartments during Pancreas Development
The pancreatic islets of Langerhans regulate glucose homeostasis. The loss of insulin-producing β cells within islets results in diabetes, and islet transplantation from cadaveric donors can cure the disease. In vitro production of whole islets, not just β cells, will benefit from a better understanding of endocrine differentiation and islet morphogenesis. We used single-cell mRNA sequencing to obtain a detailed description of pancreatic islet development. Contrary to the prevailing dogma, we find islet morphology and endocrine differentiation to be directly related. As endocrine progenitors differentiate, they migrate in cohesion and form bud-like islet precursors, or “peninsulas” (literally “almost islands”). α cells, the first to develop, constitute the peninsular outer layer, and β cells form later, beneath them. This spatiotemporal collinearity leads to the typical core-mantle architecture of the mature, spherical islet. Finally, we induce peninsula-like structures in differentiating human embryonic stem cells, laying the ground for the generation of entire islets in vitro.
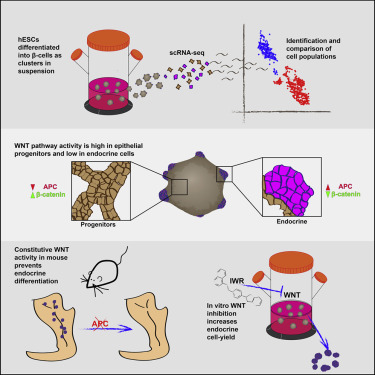
Nadav Sharon, Jordan Vanderhooft, Juerg Straubhaar, Jonas Mueller, Raghav Chawla, Quan Zhou, Elise N. Engquist, Cole Trapnell, David K. Gifford, and Doug Melton
Cell Reports. Volume 27, Issue 8, 21 May 2019, Pages 2281-2291.e5
DOI: 10.1016/j.celrep.2019.04.083
High resolution discovery of chromatin interactions
Chromatin interaction analysis by paired-end tag sequencing (ChIA-PET) is a method for the genome-wide de novo discovery of chromatin interactions. Existing computational methods typically fail to detect weak or dynamic interactions because they use a peak-calling step that ignores paired-end linkage information. We have developed a novel computational method called Chromatin Interaction Discovery (CID) to overcome this limitation with an unbiased clustering approach for interaction discovery. CID outperforms existing chromatin interaction detection methods with improved sensitivity, replicate consistency, and concordance with other chromatin interaction datasets. In addition, CID can also be applied to HiChIP data to discover chromatin interactions. We expect that the CID method will be valuable in characterizing 3D chromatin interactions and in understanding the functional consequences of disease-associated distal genetic variations.
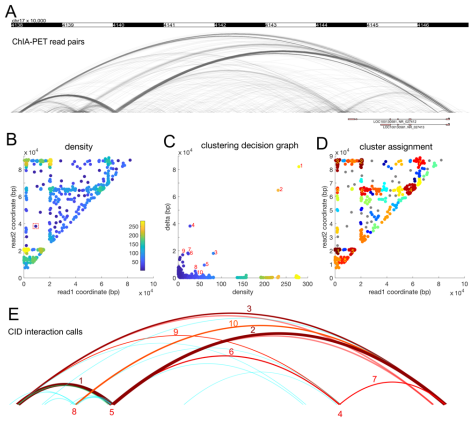
Yuchun Guo, Konstantin Krismer, Michael Closser, Hynek Wichterle, David K. Gifford
Nucleic Acids Research. Volume 47, Issue 6, 08 April 2019, Page e35
DOI: 10.1093/nar/gkz051
Disentangled Representations of Cellular Identity
We introduce a disentangled representation for cellular identity that constructs a latent cellular state from a linear combination of condition specific basis vectors that are then decoded into gene expression levels. The basis vectors are learned with a deep autoencoder model from single-cell RNA-seq data. Linear arithmetic in the disentangled representation successfully predicts nonlinear gene expression interactions between biological pathways in unobserved treatment conditions. We are able to recover the mean gene expression profiles of unobserved conditions with an average Pearson r = 0.73, which outperforms two linear baselines, one with an average r = 0.43 and another with an average r = 0.19. Disentangled representations hold the promise to provide new explanatory power for the interaction of biological pathways and the prediction of effects of unobserved conditions for applications such as combinatorial therapy and cellular reprogramming. Our work is motivated by recent advances in deep generative models that have enabled synthesis of images and natural language with desired properties from interpolation in a “latent representation” of the data.
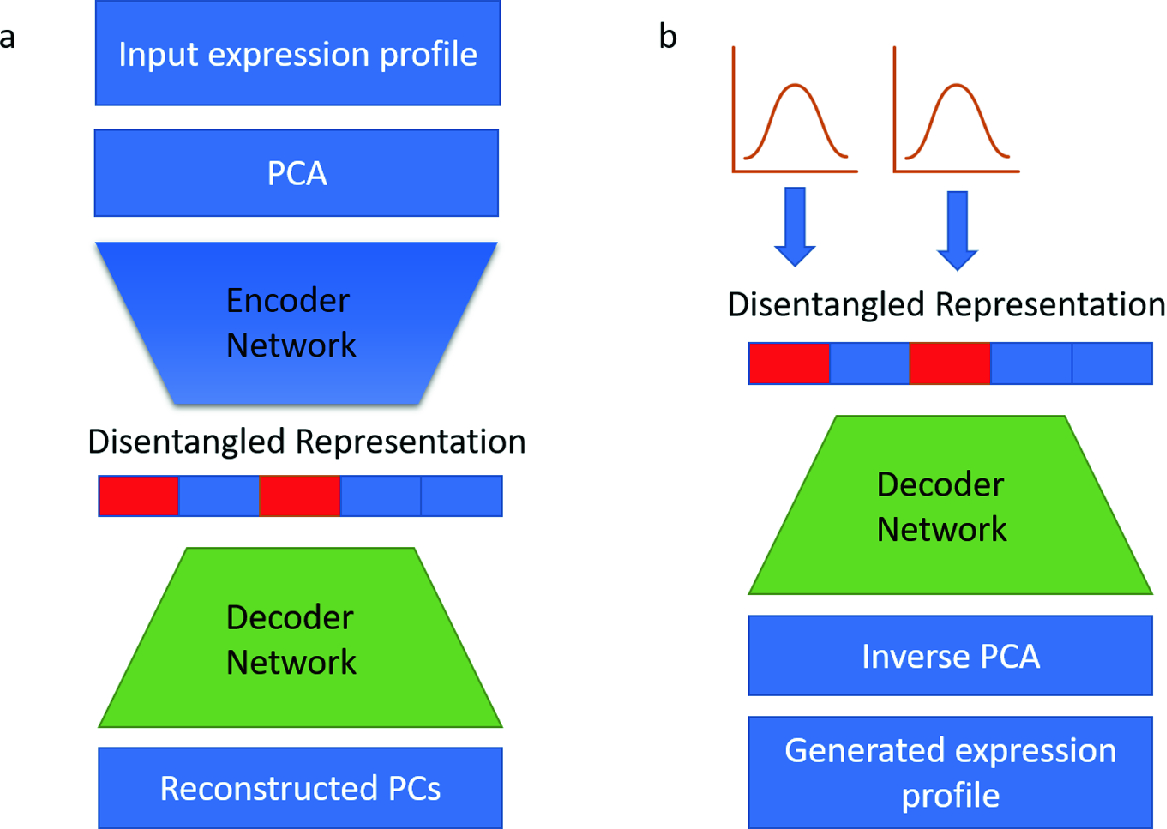
Z Wang, Yeo G, R Sherwood, and DK Gifford
Research in Computational Molecular Biology.
What made you do this? Understanding black-box decisions with sufficient input subsets
Local explanation frameworks aim to rationalize particular decisions made by a black-box prediction model. Existing techniques are often restricted to a specific type of predictor or based on input saliency, which may be undesirably sensitive to factors unrelated to the model’s decision making process. We instead propose sufficient input subsets that identify minimal subsets of features whose observed values alone suffice for the same decision to be reached, even if all other input feature values are missing. General principles that globally govern a model’s decision-making can also be revealed by searching for clusters of such input patterns across many data points. Our approach is conceptually straightforward, entirely model-agnostic, simply implemented using instance-wise backward selection, and able to produce more concise rationales than existing techniques. We demonstrate the utility of our interpretation method on various neural network models trained on text, image, and genomic data.
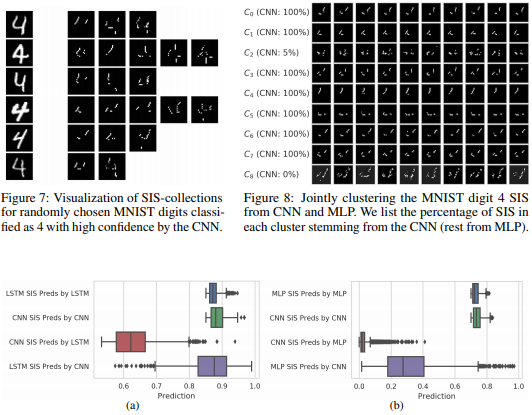
Brandon Carter, Jonas Mueller, Siddhartha Jain, and David Gifford
arXiv. preprint arXiv:1810.03805
arXiv: 1810.03805
A Peninsular Structure Coordinates Asynchronous Differentiation with Morphogenesis to Generate Pancreatic Islets
The pancreatic islets of Langerhans regulate glucose homeostasis. The loss of insulin-producing β cells within islets results in diabetes, and islet transplantation from cadaveric donors can cure the disease. In vitro production of whole islets, not just β cells, will benefit from a better understanding of endocrine differentiation and islet morphogenesis. We used single-cell mRNA sequencing to obtain a detailed description of pancreatic islet development. Contrary to the prevailing dogma, we find islet morphology and endocrine differentiation to be directly related. As endocrine progenitors differentiate, they migrate in cohesion and form bud-like islet precursors, or “peninsulas” (literally “almost islands”). α cells, the first to develop, constitute the peninsular outer layer, and β cells form later, beneath them. This spatiotemporal collinearity leads to the typical core-mantle architecture of the mature, spherical islet. Finally, we induce peninsula-like structures in differentiating human embryonic stem cells, laying the ground for the generation of entire islets in vitro.
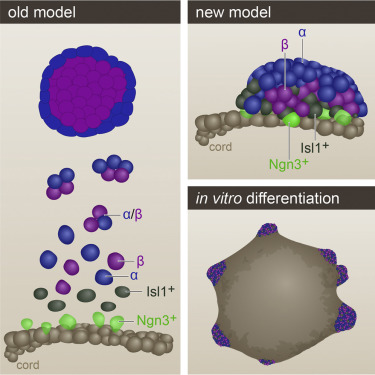
Nadav Sharon, Raghav Chawla, Jonas Mueller, Jordan Vanderhooft, Luke James Whitehorn, Benjamin Rosenthal, Mads Gürtler, Ralph R. Estanboulieh, Dmitry Shvartsman, David K. Gifford, Cole Trapnell, and Doug Melton
Cell. Volume 176, Issue 4, 7 February 2019, Pages 790-804.e13
DOI: 10.1016/j.cell.2018.12.003
2018
Predictable and precise template-free CRISPR editing of pathogenic variants
Following Cas9 cleavage, DNA repair without a donor template is generally considered stochastic, heterogeneous and impractical beyond gene disruption. Here, we show that template-free Cas9 editing is predictable and capable of precise repair to a predicted genotype, enabling correction of disease-associated mutations in humans. We constructed a library of 2,000 Cas9 guide RNAs paired with DNA target sites and trained inDelphi, a machine learning model that predicts genotypes and frequencies of 1- to 60-base-pair deletions and 1-base-pair insertions with high accuracy (r = 0.87) in five human and mouse cell lines. inDelphi predicts that 5-11% of Cas9 guide RNAs targeting the human genome are ‘precise-50’, yielding a single genotype comprising greater than or equal to 50% of all major editing products. We experimentally confirmed precise-50 insertions and deletions in 195 human disease-relevant alleles, including correction in primary patient-derived fibroblasts of pathogenic alleles to wild-type genotype for Hermansky-Pudlak syndrome and Menkes disease. This study establishes an approach for precise, template-free genome editing.
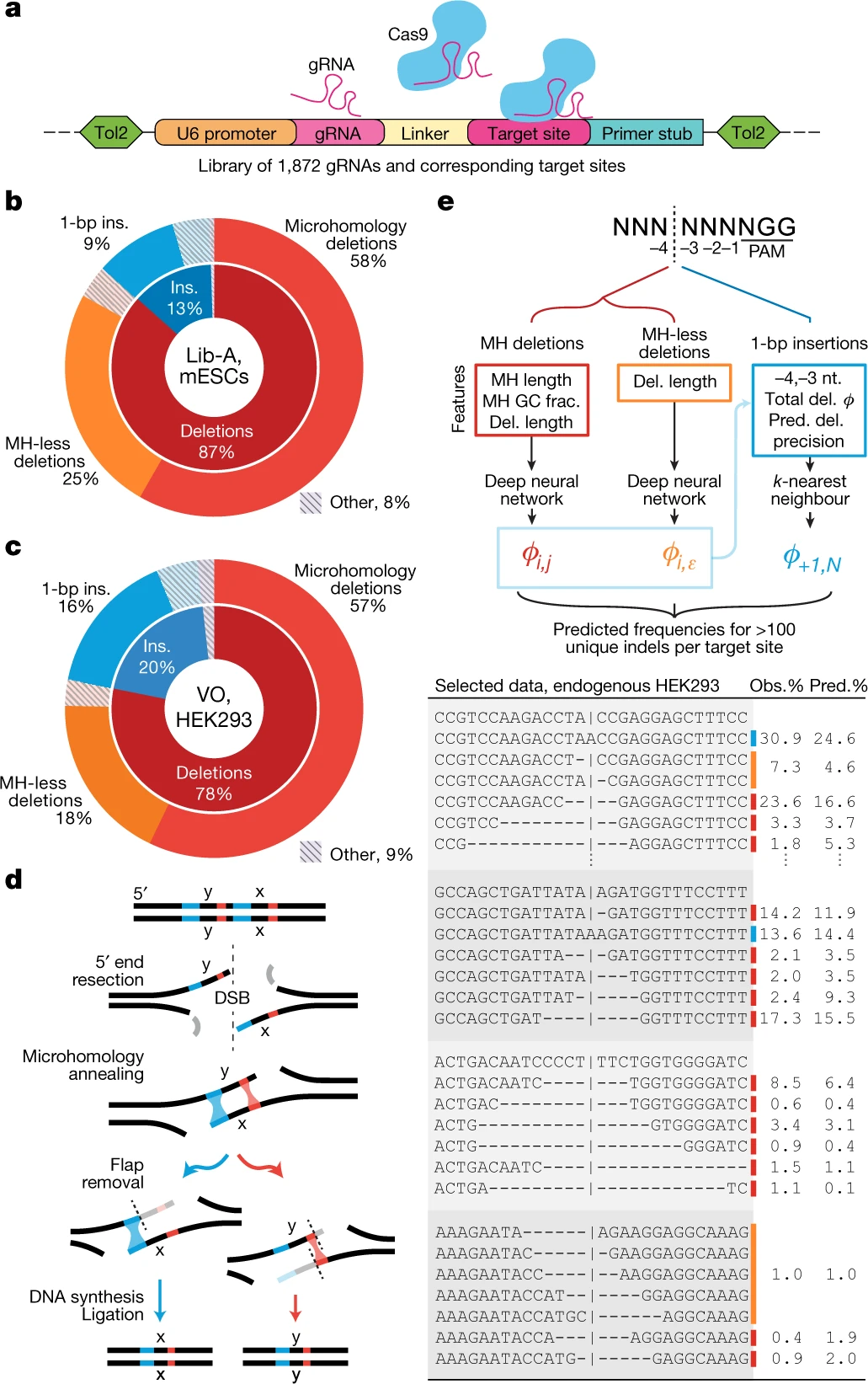
Shen MW, Arbab M, Hsu JY, Worstell D, Culbertson SJ, Krabbe O, Cassa CA, Liu DR, Gifford DK, and Sherwood RI
Nature. 563, 646–651 (2018)
DOI: 10.1038/s41586-018-0686-x
Information-based Acquisition for General Models in Bayesian Optimization
We introduce the Hilbert-Schmidt Independence Criterion (HSIC) Acquisition Function (HAF), an acquisition function for Bayesian optimization that uses HSIC to measure the statistical dependency to a distribution of interest. This enables extensions of information theoretic acquisition functions (e.g. entropy search variants) for more general models than just Gaussian Processes (GPs). HAF is also differentiable, so points can be acquired via gradient search on the input space. On a protein-DNA binding task we compare a particular instance of HAF with Thompson Sampling and Expected Reward. Though preliminary results are not impressive, we identify a major issue with the model used in this task and suggest a future direction to improve upon this work.
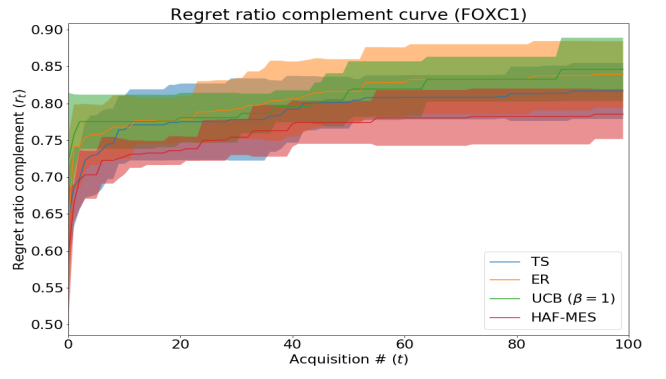
Siddhartha Jain, Nathan Hunt, and David Gifford
Bayesian Deep Learning Workshop | NeurIPS 2019.
A novel k-mer set memory (KSM) motif representation improves regulatory variant prediction
The representation and discovery of transcription factor (TF) sequence binding specificities is critical for understanding gene regulatory networks and interpreting the impact of disease-associated non-coding genetic variants. We present a novel TF binding motif representation, the k-mer set memory (KSM), which consists of a set of aligned k-mers that are over-represented at TF binding sites, and a new method called KMAC for de novo discovery of KSMs. We find that KSMs more accurately predict in vivo binding sites than position weight matrix (PWM) models and other more complex motif models across a large set of ChIP-seq experiments. Furthermore, KSMs outperform PWMs and more complex motif models in predicting in vitro binding sites. KMAC also identifies correct motifs in more experiments than five state-of-the-art motif discovery methods. In addition, KSM derived features outperform both PWM and deep learning model derived sequence features in predicting differential regulatory activities of expression quantitative trait loci (eQTL) alleles. Finally, we have applied KMAC to 1600 ENCODE TF ChIP-seq datasets and created a public resource of KSM and PWM motifs. We expect that the KSM representation and KMAC method will be valuable in characterizing TF binding specificities and in interpreting the effects of non-coding genetic variations.
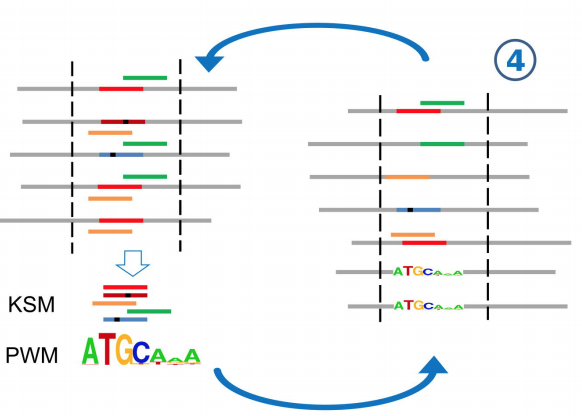
Yuchun Guo, Kevin Tian, Haoyang Zeng, Xiaoyun Guo, and David K. Gifford
Genome Research. 2018 Jun; 28(6):891-900
DOI: 10.1101/gr.226852.117
2017
-
DNase-capture reveals differential transcription factor binding modalities.
Kang D, Sherwood R, Barkal A, Hashimoto T, Engstrom L, Gifford D.
PLoS One. 2017 Dec 28;12(12):e0187046. doi: 10.1371/journal.pone.0187046. eCollection 2017. -
Working toward precision medicine: Predicting phenotypes from exomes in the Critical Assessment of Genome Interpretation (CAGI) challenges.
Daneshjou R et al.
Hum Mutat. 2017 Sep;38(9):1182-1192. doi: 10.1002/humu.23280. Epub 2017 Jul 7. -
Sequence to Better Sequence: Continuous Revision of Combinatorial Structures
Mueller J, Gifford DK, Jaakkola T.
International Conference on Machine Learning. pp. 2536-2544. 2017. -
Modeling Persistent Trends in Distributions
Mueller J, Jaakkola T, Gifford DK.
Journal of the American Statistical Association. 2017 -
Differential chromatin profiles partially determine transcription factor binding
Chen R and Gifford DK.
PLoS One. 2017 Jul 13;12(7):e0179411. doi: 10.1371/journal.pone.0179411. eCollection 2017. -
Predicting the impact of non-coding variants on DNA methylation
Zeng H and Gifford DK.
Nucleic Acids Res. 2017 Jun 20;45(11):e99. doi: 10.1093/nar/gkx177. -
Accurate eQTL prioritization with an ensemble-based framework
Zeng H, Edwards MD, Guo Y, Gifford DK.
Hum Mutat. 2017 Feb 21, doi: 10.1002/humu.23198. -
Predicting gene expression in massively parallel reporter assays: A comparative study
Kreimer A, Zeng H, Edwards MD, Guo Y, Tian K, Shin S, Welch R, Wainberg M, Mohan R, Sinnott-Armstrong NA, Li Y, Eraslan G, Amin TB, Goke J, Mueller NS, Kellis M, Kundaje A, Beer MA, Keles S, Gifford DK, Yosef N.
Hum Mutat. 2017 Feb 21., doi: 10.1002/humu.23197. -
Modular combinatorial binding among human trans-acting factors reveals direct and indirect factor binding
Guo Y, Gifford DK.
BMC Genomics. 2017 Jan 6;18(1):45., doi: 10.1186/s12864-016-3434-3.
2016
-
Expression of Terminal Effector Genes in Mammalian Neurons Is Maintained by a Dynamic Relay of Transient Enhancers
Rhee HS, Closser M, Guo Y, Bashkirova EV, Tan CG, Gifford DK, Wichterle H.
Neuron. 2016 Dec 21;92(6):1252-1265., doi: 10.1016/j.neuron. 2016.11.037. -
Identification of new branch points and unconventional introns in Saccharomyces cerevisiae
Gould GM, Paggi JM, Guo Y, Phizicky DV, Zinshteyn B, Wang ET, Gilbert WV, Gifford DK, Burge CB.
RNA. 2016 Oct;22(10):1522-34., doi: 10.1261/ma.057216.116. -
A Synergistic DNA Logic Predicts Genome-wide Chromatin Accessibility
Hashimoto TB, Sherwood RI, Kang DD, Barkal AA, Zeng H, Emons BJM, Srinivasan S, Rajagopal N, Jaakkola T, and Gifford DK.
Genome Research, doi: 10.1101/gr.199778.115 -
Learning Population-Level Diffusions with Generative Recurrent Networks
Hashimoto TB, Gifford DK, Jaakkola TS.
Proceedings of the 33rd International Conference on Machine Learning (ICML). in PMLR 48:2417-2426 (2016). -
Convolutional neural network architectures for predicting DNA-protein binding
Zeng H, Edwards MD, Liu G, Gifford DK.
Bioinformatics. 2016 Jun 15;32(12):i121-i127. doi: 10.1093/bioinformatics/btw255. -
A distant trophoblast-specific enhancer controls HLA-G expression at the maternal-fetal interface
Ferreira LM, Meissner TB, Mikkelsen TS, Mallard W, O’Donnell CW, Tilburgs T, Gomes HA, Camahort R, Sherwood RI, Gifford DK, Rinn JL, Cowan CA, Strominger JL.
Proc Natl Acad Sci U.S.A . 2016 May 10;113(19):5364-9. doi: 10.1073/pnas.1602886113. -
Cas9 Functionally Opens Chromatin Barkal AA
Srinivasan S, Hashimoto T, Gifford DK, Sherwood RI.
PLoS One. 2016 Mar 31;11(3):e0152683. -
High-throughput mapping of regulatory DNA
Rajagopal, Nisha, Sharanya Srinivasan, Kameron Kooshesh, Yuchun Guo, Matthew D. Edwards, Budhaditya Banerjee, Tahin Syed, Bart JM Emons, David K. Gifford, and Richard I. Sherwood.
Nature Biotechnology. 34, 167–174 (2016)
2015
-
GERV: a statistical method for generative evaluation of regulatory variants for transcription factor binding
H. Zeng, T. Hashimoto, D.D. Kang, D.K. Gifford.
Bioinformatics. 32 (4): 490-496 (2015) -
High resolution mapping of enhancer-promoter interactions
Christopher Reeder, Michael Closser, Huay Mei Poh, Kuljeet Sandhu,Hynek Wichterle, David Gifford.
PLOS ONE. doi:10.1371/journal.pone.0122420 May 13, 2015
2014
-
Long-term persistence and development of induced pancreatic beta cells generated by lineage conversion of acinar cells
Weida Li, Claudia Cavelti-Weder, Yinying Zhang, Kendell Clement, Scott Donovan, Gabriel Gonzalez, Jiang Zhu, Marianne Stemann, Ke Xu, Tatsu Hashimoto, Takatsugu Yamada, Mio Nakanishi, Yuemei Zhang, Samuel Zeng, David Gifford, Alexander Meissner, Gordon Weir, and Qiao Zhou.
Nat Biotechnol. 2014 Dec;32(12):1223-30. doi: 10.1038/nbt.3082. Epub 2014 Nov 17 -
Gene co-regulation by Fezf2 selects neurotransmitter identity and connectivity of corticospinal neurons
S. Lodato, B.J. Molyneaux, E. Zuccaro, L.A. Goff, H.H. Chen, W. Yuan, A. Meleski, E. Takahashi, S. Mahony, J.L. Rinn, D.K., P. Arlotta.
Nat Neurosci. 2014 Aug;17(8):1046-54. doi: 10.1038/nn.3757. Epub 2014 Jul 6 -
Interactions between chromosomal and nonchromosomal elements reveal missing heritability
Matthew D. Edwards, Anna Symbor-Nagrabska, Lindsey Dollard, David K. Gifford, Gerald R. Fink.
Proc. Natl. Acad. Sci U.S.A., 2014, May 13. pii: 201407126 -
An integrated model of multiple-condition ChIP-Seq data reveals predeterminants of Cdx2 binding”
S. Mahony, M. D. Edwards, E. O. Mazzoni, R. I. Sherwood, A. Kakumanu, C. A. Morrison, H. Wichterle, D. K. Gifford .
PLoS Comput Biol. 2014 Mar 27;10(3):e1003501. doi: 10.1371/journal.pcbi.1003501. eCollection 2014 Mar -
Universal count correction for high-throughput sequencing
T.B. Hashimoto, M. D. Edwards, D. K. Gifford
PLoS Comput Biol. 2014 Mar 6;10(3):e1003494. doi: 10.1371/journal.pcbi.1003494. eCollection 2014 -
MARIS: method for analyzing RNA following intracellular sorting
S. Hrvatin, F. Deng, C. W. O’Donnell, D. K. Gifford, D. A. Melton.
PLoS One. 2014 Mar 3;9(3):e89459. doi: 10.1371/journal.pone.0089459. eCollection 2014 -
Differentiated human stem cells resemble fetal, not adult, ß cells.
S. Hrvatin, C. W. O’Donnell, F. Deng, J. R. Millman, F. W. Pagliuca, P. DiIorio, A. Rezania, D. K. Gifford, D. A. Melton.
Proc Natl Acad Sci U.S.A., 2014 Feb 25;111(8):3038-43. doi: 10.1073/pnas.1400709111. Epub 2014 Feb 10 -
Discovery of directional and nondirectional pioneer transcription factors by modeling DNase profile magnitude and shape
R. I. Sherwood, T. Hashimoto, C. W. O’Donnell, D. Lewis, A. A. Barkal, J. P. van Hoff, V. Karun, T. Jaakkola, D. K. Gifford.
Nat Biotechnol. 2014 Feb;32(2):171-8. doi: 10.1038/nbt.2798. Epub 2014 Jan 19
2013
-
A Cdx4-Sall4 Regulatory Module Controls the Transition from Mesoderm Formation to Embryonic Hematopoiesis
E.J. Paik, S. Mahony, R. M. White, E.N. Price, A. Dibiase, B. Dorjsuren, C. Mosimann, A. J. Davidson, D. Gifford, L. I. Zon.
Stem Cell Reports. 2013 Nov 7;1(5):425-436 -
Saltatory remodeling of Hox chromatin in response to rostrocaudal patterning signals
E. O. Mazzoni, S. Mahony, M. Peljto, T. Patel, S. R. Thornton, S. McCuine, C. Reeder, L. A. Boyer, R. A. Young, D. K. Gifford, H. Wichterle
Nat Neurosci. 2013 Sep;16(9):1191-8. doi: 10.1038/nn.3490. Epub 2013 Aug 18 -
Neuroscience. Mapping neuronal diversity one cell at a time
H. Wichterle, D. Gifford, E. Mazzoni
Science. 2013 Aug 16;341(6147):726-7. doi: 10.1126/science.1235884. -
Synergistic binding of transcription factors to cell-specific enhancers programs motor neuron identity E.O. Mazzoni, S. Mahony, M. Closser, C. A. Morrison, S. Nedelec, D. J. Williams, D. An, D. K. Gifford, H. Wichterle Nat Neurosci. 2013 Sep;16(9):1219-27. doi: 10.1038/nn.3467. Epub 2013 Jul 21
-
High Resolution Modeling of Chromatin Interactions
C. Reeder, D. Gifford
Research in Computational Molecular Biology, 186-198, 2013 -
A multi-parametric flow cytometric assay to analyze DNA-protein interactions
M. Arbab, S. Mahony, H. Cho, J. M. Chick, P. A. Rolfe, J. P. van Hoff, V. W. Morris, S. P. Gygi, R. L. Maas, D. K. Gifford, R. I. Sherwood
Nucleic Acids Res. 2013 Jan. 41(2)
2012
-
Global gene deletion analysis exploring yeast filamentous growth
O. Ryan, R. S. Shaprio, C. F. Kurat, D. Mayhew, A. Baryshnikova, B. Chin, Z. Y. Lin, M. J. Cox, F. Vizeacoumar, D. Cheung, S. Bahr, K. Tsui, F. Tebbji, A. Sellam, F. Istel, T. Schwarzmuller T, T. B. Reynolds, K. Kuchler, D. K. Gifford, M. Whiteway, G. Giaever, C. Nislow, M. Costanzo, A. C. Gingras, R. D. Mitra, B. Andrews, G. R. Fink, L. E. Cowen, C Boone
Science, 2012 Sep 14’337(6100):1353-6. -
High resolution genome wide binding event finding and motif discovery reveals transcription factor spatial binding constraints.
Y. Guo, S. Mahony, D. K. Gifford.
PLoS Comput Biol. 2012 Aug;8(8):e1002638. -
Ruler arrays reveal haploid genomic structural variation
P. A. Rolfe, D. A. Bernstein, P. Grisafi, D. K. Gifford
PLoS One, 2012;7(8):e43210 -
Lineage-based identification of cellular states and expression programs
T. Hashimoto, T. Jaakkola, R. Sherwood, E.O. Mazzoni, H. Wichterle, D. Gifford
Bioinformatics, 2012 Jun 15;28(12):i250-7 -
High Resolution genetic mapping with pooled sequencing
M. D. Edwards, D. K. Gifford
BMC Bioinformatics, 2012 Apr 19l13 Suppl 6:S8
2011
-
Embryonic stem cell-based mapping of developmental transcriptional programs
E. O. Mazzoni, S. Mahony, M. Iacovino, C. A. Morrison, G. Mountoufaris, M. Closser, W. A. Whyte, R. A. Young, M. Kyba, D. K. Gifford, H. Wichterle H.
Nat Methods, 2011 Nov 13;8(12):1056-8. doi: 10.1038/nmeth.1775 -
ReadDB provides efficient storage for mapped short reads
P. A. Rolfe, D. K. Gifford
BMC Bioinformatics, 2011 Jul 7;12:278. -
Discovering regulatory overlapping RNA transcripts
T. Danford, R. Dowell, S Agarwala, P. Grisafi, G. Fink, D. Gifford
J Comput Biol., 2011 Mar;18(3):295-303 -
Ligand-dependent dynamics of retinoic acid receptor binding during early neurogenesis
S. Mahony, E. O. Mazzoni, S. McCuine, R. A. Young, H. Wichterle, D. K.Gifford.
Genome Biol., 2011;12(1):R2. Epub 2011 Jan 13
2010
-
Rapid haplotype inference for nuclear families
A. L. Williams, D. E. Housman, M. C. Rinard, D. K. Gifford
Genome Biol., 2010;11(10):R108. Epub 2010 Oct 29 -
Discovering homotypic binding events at high spatial resolution
Y. Guo, G. Papachristoudis, R. C. Altshuler, G. K. Gerber, T. S. Jaakkola, D. K. Giffo, S. Mahony
Bioinformatics, 2010 Dec 15;26(24):3028-34. Epub 2010 Oct 21 -
Global control of motor neuron topography mediated by the repressive actions of a single hox gene
H. Jung, J. Lacombe, E.O. Mazzoni, K. F. Liem Jr, J. Grinstein, S. Mahony, D. Mukhopadhyay, D. K. Gifford, R. A. Young, K. V. Anderson, H. Wichterle, J. S. Dasen
Neuron, 2010 Sep 9;67(5):781-96 -
Control of transcription by cell size
C. Y. Wu, P. A. Rolfe, D. K. Gifford
PLoS Biol., 2010 Nov 2;8(11):e1000523 -
Genotype to Phenotype: A Complex Problem
R. D. Dowell, O. Ryan, A. Jansen, D. Cheung, S. Agarwala, T. Danford, D. A. Bernstein, P. A. Rolfe, L. E. Heisler, B. Chin, C. Nislow, G. Giaever, P. C. Phillips, G. R. Fink, D. K. Gifford, and C. Boone
Science , 23, April, 2010, p. 469 -
Feed-forward Regulation of a Cell Fate Determinant by an RNA-binding Protein Generates Asymmetry in Yeast
J. Wolff, R. D. Dowell, S. Mahony, M. Rabani, D. K. Gifford, and G. R. Fink.
Genetics 2010
2009 and Earlier
-
Toggle involving cis-interfering noncoding RNAs controls variegated gene expression in yeast
S. L. Bumgarner, R. D. Dowell, P. Grisafi, D. K. Gifford, and G. R. Fink
PNAS 106(43), October, 2009, pp18321-18326 -
Analysis of the mouse embryonic stem cell regulatory networks obtained by ChIP-chip and ChIP-PET
D. Mathur, T. W. Danford, L. A. Boyer, R. A. Young, D. K. Gifford, and R. Jaenisch
Genome Biol., 2008;9(8) -
Tissue-specific transcriptional regulation has diverged significantly between human and mouse
D. T. Odom, R. D. Dowell, E. S. Jacobsen, W. Gordon, T. W. Danford, K. D. MacIsaac, P. A. Rolfe, C. M. Conboy, D. K. Gifford, and E. Fraenkel
Nature Genetics, 39:6, 730-732, June, 2007 -
Automated Discovery of Functional Generality of Human Gene Expression Programs
G. K. Gerber, R. D. Dowell, T. S. Jaakkola, and D. K. Gifford
PLOS Computational Biology, 3:8, August 2007 -
Semi-supervised analysis of gene expression profiles for lineage-specific development in the Caenorhabditis elegans embryo
Y. Qi, P. E. Missiuro, A. Kapoor, C. P. Hunter, T. S. Jaakkola D. K. Gifford,and H. Ge
Bioinformatics, 15;22(14), July 2006, pp. 417-423 -
Control of developmental regulators by Polycomb in human enbryonic stem cells
T. I. Lee, R. G. Jenner, L. A. Boyer, M. G. Guenther, S. S. Levine, R. M Kumar, B. Chevalier, S. E. Johnstone, M. F. Cole, K. Isono, H. Koseki, T. Fuchikami, K. Abe, H. L. Murray, J. P. Zucker, B. Yuan, G. W. Bell, E. Herbolsheimer, N. M. Hannett, K. Sun, D. T. Odom, A. P. Otte, T. L. Volkert, D. P. Bartel, D. A. Melton, D. K. Gifford, R. Jaenisch, and R. A. Young
Cell, 125(2), April, 2006, pp 301-313. -
An improved map of conserved regulatory sties for Saccharomyces cerevisiae
K. D. MacIsaac, T. Wang, D. B. Gordon, D. K. Gifford, G. D. Stormo, and E. Fraenkel
BMC Bioinformatics, March, 2006 -
A hypothesis-based approach for identifying the binding specificity of regulatory proteins from chromatin immunoprecipitation data
K. D. MacIsaac, D. B. Gordon, L. Nekludova, D. T. Odom, J. Schreiber, D. K. Gifford, R. A. Young, and E. Fraenkel
Bioinformatics, Feb., 2006. -
Polycomb complexes repress developmental regulators in murine embryonic stem cells
L. A. Boyer, K. Plath, J. Zeitlinger, T. Brambrink, L. A. Medeiros, T. I Lee., S. S. Levine, M. Wernig, A. Tajonar, M. K. Ray, G. W. Bell, A. P. Otte, M. Vidal, D. K. Gifford, R. A. Young, and R. Jaenisch.
Nature, 441:349-353, May 2006 -
Coordinated binding of NF-kB family members in the response of human cells to lipopolysaccharide
J. Schreiber, R. G. Jenner, H. L. Murray, G. K. Gerber, D. K. Gifford and R. A. Young
Proceedings of the National Academy of Sciences (PNAS), 103(10):5899-5904, 2006 -
High-resolution computational models of genome binding events
A. Qi, P.A. Rolfe, K. MacIsaac, G.K. Gerber, D. Pokholok, J. Zeitlinger, T. Danford, R.D. Dowell, E. Fraenkel, T.S. Jaakkola, R.A. Young, and D.K. Gifford
Nature Biotechnology, 24, 963-960 (2006) [Supplemental material] [software] -
Core Transcriptional Regulatory Circuitry in Human Hepatocytes.
D.T. Odom, R.D. Dowell, E.S. Jacobsen, L. Nekludova, P.A. Rolfe, T.W. Danford, D.K. Gifford, E. Fraenkel, G.I. Bell, and R.A. Young.
Nature/EMBO Molecular Systems Biology, msb4100059, 2 May 2006. -
Genome-wide map of nucleosome acetylation and methylation in yeast
D. K. Pokholok, C. T. Harbison, S. Levine, M. Cole, N. M. Hannett, T. I. Lee, G. W. Bell, K. Walker, P. A. Rolfe, E. Herbolsheimer, J. Zeitlinger, F. Lewitter, D. K. Gifford, and R. A. Young
Cell, 122(4), August, 2005. pp. 517-527. -
Core Transcriptional Regulatory Circuitry in Human Embryonic Stem Cells
L. A. Boyer, T. I. Lee, M. F. Cole, S. E. Johnstone, S. S. Levine, J. P. Zucker, M. G. Guenther, R. M. Kuman, H. L. Murray, R. G. Jenner, D. K. Gifford, D. A. Melton , R. Jaenisch, and R. A. Young
Cell, Vol. 122, 1-20, September, 2005 -
Global postition and recruitment of HATS and HDACS in the yeast genome
F. Robert, D.K. Pokholok, N. M Hannett, N. J. Rinaldi, M. Chandy, A. Rolfe, J. L. Workman, D. K. Gifford, and R. A. Young
Mol. Cell, 16(2), October, 2004, pp. 199-209 -
Deconvolving cell cyle expression data with complementary information
Z. Bar-Joseph, S. Farkash, D. K. Gifford, I. Simon, R. Rosenfeld
Bioinformatics, Vol. 20 Suppl. 1, 2004. pp i23-i30 -
Transcriptional regulatory code of a eukaryotic genome
C. Harbison, D. B. Gordon, T. I Lee, N. J. Rinaldi, K. D. MacIsaac, T. W. Danford, N. M. Hannett, J.B. Tagne, D. B. Reynolds, J. Yoo, E. G. Jennings, J. Zeitlinger, D. K. Pokholok, M. Kellis, P. A. Rolfe, K. T. Takusagawa, E. S. Lander, D. K. Gifford, E. Fraenkel, and R. A. Young
Nature, 431:99-104, September, 2004 -
Control of Pancreas and Liver Gene Expression by HNF Transcription Factors.
Odom, D. T., Zizlsperger, N., Gordon, D. B., Bell, G. W., Rinaldi, N. J., Murray, H. L., Volkert, T. L., Schreiber, J., Rolfe, P. A., Gifford, D. K., Fraenkel, E., Bell, G. I., Young, R. A.
Science, 303:1378-1381, February, 2004 -
Comparing the Continuous Representation of Time Series Gene Expression Profiles to Identify Differentially Expressed Genes
Z. Bar-Joseph, G.K. Gerber, I. Simon, D.K. Gifford, and T.S. Jaakkola
Proceedings of the National Academy of Sciences, 2003 Sept. 2; 100(18):10146-10151 -
Negative Information for Motif Discovery
Takusagawa, K. T., Gifford, D. K.
Pacific Symposium on Biocomputing, 9:360-371, 2004 -
Computational discovery of gene modules and regulatory networks
Bar-Joseph, Z., Gerber, G. K., Lee, T. I., Rinaldi, N. J., Yoo, J. Y., Robert, F., Gordon, D. B., Fraenkel, E., Jaakkola, T. S., Young, R. A., Gfford D. K.
Nature Biotechnology, 21, pp. 1337-1342 November, 2003 -
Continuous Representations of Time Series Gene Expression Data
Z. Bar-Joseph, Gerber, D. Gifford, T Jaakkola and I. Simon G. Gerber, D. Gifford, T. Jaakkola and I. Simon.
J Comput Biol., 2003;10(3-4):341-56 -
K-ary Clustering with Optimal Leaf Ordering for Gene Expression Data
Ziv Bar-Joseph, Erik D. Demaine, David K. Gifford, Angèle M. Hamel, Tommy S. Jaakkola and Nathan Srebro
Bioinformatics, Vol. 19, No. 9, 2003 -
Transcriptional Regulatory Networks In Saccharomyces cerevisiae
T.I. Lee, N. J. Rinaldi, F. Robert, D. T. Odom, Z. Bar-Joseph, G. K. Gerber, D. K Gifford and R. A. Young
Science, 298:799-804 (2002) -
K-ary Clustering with Optimal Leaf Ordering for Gene Expression Data
Ziv Bar-Joseph, Erik D. Demaine, David K. Gifford, Angèle M. Hamel, Tommi S. Jaakkola and Nathan Srebro
Proceedings of the 2nd Workshop on Algorithms in Bioinformatics (WABI 2002), Rome, Italy, September 17-11 -
Combining Location and Expression Data for Principled Discovery of Genetic Regulatory Network Models.
Alexander J. Hartemink, David K. Gifford, Tommi S. Jaakkola, and Richard A. Young
Pacific Symposium on Biocomputing, 2002, Kauai, January 2002 -
Bayesian Methods for Elucidating Genetic Regulatory Networks
Hartemink, A. J., Gifford, D. K., Jaakkola, T. S., Young, R. A.
IEEE Intelligent Systems in Biology, Vol. 17, No. 2, March, 2002, pp. 37-43 -
Serial Regulation of Transcriptional Regulators in the Yeast Cell Cycle
Simon, I., Barnett, J., Hannett, N., Harbison, C. T., Rinaldi, N. J., Volkert, T. L. Wyrick, J. J., Zeitlinger, J., Gifford., D. K., Jaakkola, T. S., Young, R. A.
Cell, 106, Sept., 2001, p. 667-708 -
A new approach to analyzing gene expression time series data.
Z. Bar-Joseph, G. Gerber, D. Gifford, T. Jaakkola and I. Simon
Proceedings of The Sixth Annual International Conference on Research in Computational Molecular Biology (RECOMB), 2002, pp 39-48 -
Blazing pathways through genetic mountains
Gifford, D. K.
Science, 2001 Sep 14;293(5537):2049-51 -
Fast optimal leaf ordering for hierarchical clustering.
Z. Bar-Joseph, D. Gifford, and T. Jaakkola
Bioinformatics (Proceedings of ISMB 2001), 17(S1), 2001, pp 22-19 -
Using Graphical Models and Genomic Expression Data to Statistically Validate Models of Genetic Regulatory Networks
Alexander J. Hartemink, David K. Gifford, Tommi S. Jaakkola, and Richard A. Young
Pacific Symposium on Biocomputing, 2001, Hawaii, January 2001 -
Maximum Likelihood Estimation of Optimal Scaling Factors for Expression Array Normalization
Alexander J. Hartemink, David K. Gifford, Tommi S. Jaakkola, and Richard A. Young
SPIE BiOS 2001, San Jose, California, January 2001 -
Experimental Efficiency of Programmed Mutagenesis
Julia Khodor and David K. Gifford
New Generation Computing 20:3, pp 307-315, 2002 -
Programmed Mutagenesis is Universal
Julia Khodor, and David K. Gifford
Theory of Computing Systems, 255, pp 483-499, 2002. -
Simulating Biological Reactions: A Modular Approach
Alexander J. Hartemink, Tarjei S. Mikkelsen, and David K. Gifford 5th Annual
DIMACS Workshop on DNA-Based Computers, Boston, Massachusetts, June 1999 -
Automated Constraint-Based Nucleotide Sequence Selection for DNA Computation
Alexander J. Hartemink, David K. Gifford, and Julia Khodor
4th Annual DIMACS Workshop on DNA-Based Computers, Philadelphia, Pennsylvania, June 1998 -
Design & Implementation of Computational Systems Based on Programmed Mutagenesis
Khodor, J. and Gifford, D. K.
DIMACS Workshop on Nucleic Acid Selection and Computing, Princeton University, March, 1998 -
Thermodynamic Simulation of Deoxyoligonucleotide Hybridization for DNA Computation
Alexander J. Hartemink and David K. Gifford
3rd Annual DIMACS Workshop on DNA-Based Computers, Philadelphia, Pennsylvania, June 1997 -
The Efficiency of Sequence-Specific Separation of DNA Mixtures for Biological Computing
Julia Khodor and David K. Gifford
3rd Annual DIMACS Workshop on DNA-Based Computers, Philadelphia, Pennsylvania, June 1997